Application Exam Questions
Application Exam Questions
- Bioinformatics and computational biology are huge and growing areas of active research with a dynamic array of subspecialities - phylogenetic analysis has advanced in leaps and bounds with the availablity of molecular data, genomic-, transcriptomic-, proteomic and other cross-sectional views are slowly beginning to unravel some of the intricacies of the cell's inner workings, predictive models for molecular medicine and bioengineering may help shape our society's future. These tasks are what motivates bioinformatics worldwide, seeking to develp novel ways to reason about biology.
2003 - Consequences of Homology
A bacterial genome has been sequenced. Comparison with tRNA synthetase COGs has failed to turn up an annotation for a Glutaminyl tRNA synthetase gene. Assume that the genome sequence is complete, has been assembled without frameshift errors and all gene models are correct. The sequence for Glutaminyl tRNA synthetase has not simply been overlooked. Answer the following questions briefly.
- What is a COG ?
- State two reasons why the endpoint of a biochemical pathway could be present in an organism but a gene on the pathway would fail to be found in a COG analysis.
- Briefly(!) state what wet-lab experiments you would propose to confirm (or disprove) the hypotheses you have stated above ?
Comment
2003 - Domains and Homology
- Briefly define homologs, orthologs and paralogs.
- Are the proteins depicted above homologs ?
- Briefly explain the contents, implications and use of this CDART entry.
(I would consider the words "contents, implications and use" to be too vague for a future exam question.)
2003 - Homology
The COGs database employs a definition of orthologs that is based on reciprocal highest similarity.
- Briefly explain the principle and how it distinguishes orthologs from paralogs.
You have built a homology model based on the pairwise alignment of a C. elegans Glutaminyl-tRNA synthetase sequence to a template that you have identified with a BLAST search. Upon analysis of your model structure, you note that the presumed ATP-binding site of the model appears to be blocked by an arginine sidechain.
- Briefly define the key steps involved in building a homology model.
- Based on these steps, identify two probable sources of the discrepancy between the model and the function of the protein.
- Suggest a bioinformatics strategy to improve your model, given the problems you have identified above.
2003 - Expression Analysis
In order to study the coordination of gene expression in the yeast cell cycle, you have created a synchronized, growing culture of Saccharomyces cerevisiaea cells and harvested mRNA at ten successive time points along one replication cycle. You now plan to perform two-color microarray (spotted array) experiments.
- Describe the principle and key steps of this experiment, from mRNA samples to a series of scanned images.
- Describe the key steps involved in going from a scanned image to expression profiles of individual genes.
- After clustering genes according to similarity of expression profiles, you will have sets of genes with correlated expression profiles. You hypothesize that these may be coregulated genes. What bioinformatics procedure(s) can you suggest to help you annotate shared functions for your clusters of genes ?
- What bioinformatics procedure(s) can you suggest to pursue the question whether these genes may be coregulated ?
2003 - Integrated processes
Read the following abstract:
- Structure of TCTP reveals unexpected relationship with guanine nucleotidefree chaperones
- Paul Thaw, Nicola J. Baxter, Andrea M. Hounslow, Clive Price, Jonathan P. Waltho and C. Jeremy Craven: Nature Struct Biol 8: 701–704 (2001)
- The translationally controlled tumor-associated proteins (TCTPs) are a highly conserved and abundantly expressed family of eukaryotic proteins that are implicated in both cell growth and the human acute allergic response but whose intracellular biochemical function has remained elusive. We report here the solution structure of the TCTP from Schizosaccharomyces pombe, which, on the basis of sequence homology, defines the fold of the entire family. We show that TCTPs form a structural superfamily with the Mss4/Dss4 family of proteins, which bind to the GDP/GTP free form of Rab proteins (members of the Ras superfamily) and have been termed guanine nucleotide-free chaperones (GFCs). Mss4 also acts as a relatively inefficient guanine nucleotide exchange factor (GEF). We further show that the Rab protein binding site on Mss4 coincides with the region of highest sequence conservation in the TCTP family. This is the first link to any other family of proteins that has been established for the TCTP family and suggests the presence of a GFC/GEF at extremely high abundance in eukaryotic cells.
This abstract reports several pieces of data and mentions several pieces of prior information.
- List the most important data and information entities and databases that have been used in this study.
- Summarize on approximately one-half page or less the essential steps of how these entities were related to each other in this study. You may use any representation that is reasonable such as pseudocode, a flowchart, or other type of sketch.
Note that you are not required to understand the biochemical processes that are described here, nor are you required to comment on the cell-biological implications. One of the key steps has been underlined by me - you must understand how such a conclusion can be drawn in the situation that is described. You are to summarize the flow of data: the entities that are being referred to, and the experimental and computational procedures.
2003 - Integrated processes
Read the following abstract:
- Insights into DNA recombination from the structure of a RAD51-BRCA2 complex.
- Pellegrini L, Yu DS, Lo T, Anand S, Lee M, Blundell TL, Venkitaraman AR.: Nature 420:287-293 (2002)
- In this landmark paper on the breast cancer susceptibility gene BRCA2, the authors provide an intriguing mechanistic link with RAD51, a recombinase that plays an essential role in DNA repair by facilitating homologous recombination of intact and damaged DNA strands. Sequence repeats in the BRCA2 gene were reported as early as 1996 and have allowed the authors to define and express an isolated repeat domain (BRC4). It was known that the sequence repeats mediate binding of BRCA2 to RAD51. Sequence similarity (30 % identity) between RAD51 and E. coli RecA had allowed to define and express a domain of RAD51. The BRC4-RAD51 complex was prepared, crystallized, and its structure was solved at high resolution. The crystal structure shows extensive specific interactions between BRC4 and RAD51, additionally it demonstrates structural similarity between RAD51 and RecA, supporting the idea of a shared mechanism. Two crucial insights emerge: firstly, the authors are able to map breast cancer associated mutations of BRCA2 (from the NIH Breast Cancer Information Core database) to specific BRC4 residues that appear to have critical roles for RAD51 binding. As a general rule: cancer associated mutations appear to disrupt interactions that seem to be critical for binding. And secondly, the mode of interaction between BRC4 and RAD51 allows to hypothesize about the function of the repaets. RecA forms helical nucleoprotein filaments as part of its function. Superposition of the RAD51 structure on the model of the RecA filament thus allows to model the interactions of RAD51 domains in a RAD51 filament. Comparison with the BRC4 complex structure shows that BRC4 binds RAD51 in a location that would be occupied by another RAD51 molecule, in a RAD51 helical filament. Furthermore, the conformation of BRC4 in this epitope mimics the conformation of the RAD51 epitope it apparently displaces, as well, the BRC consensus sequence motif of this epitope (
GFXTASG
) is similar to the highly conserved sequence of this epitope in RAD51 homologues (GFTTATE
).
- List briefly they key entities (models, abstractions, observations, database resources ... ) that have been used in this analysis.
- Sketch the key bioinformatic procedures with which these entities were related to each other in this study. You may use any representation that is reasonable such as a Data Flow Diagram, or other type of sketch, or a description in pseudocode.
2003 - Phylogenetic analysis
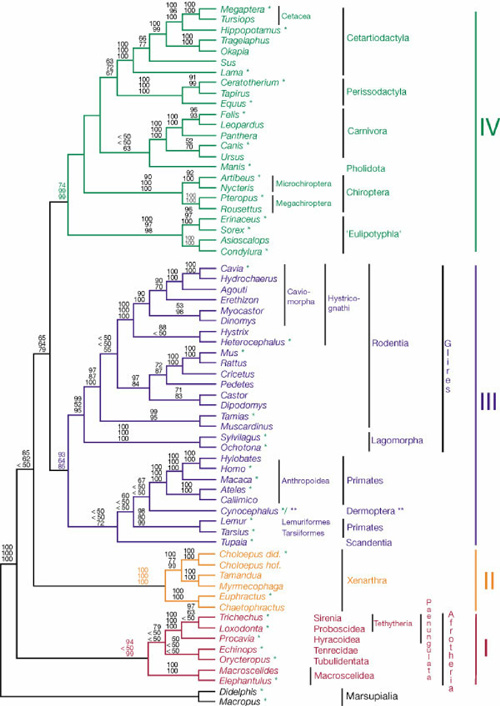
- Briefly explain the principles of this graph in terms of what can be observed, what must be inferred and how this graph integrates this information.
- What do the numbers among the branches mean ?
- Which parts of this graph correspond to the following terms:
- Clade
- Bifurcation
- Root
- Briefly state the principle of maximum parsimony and briefly explain how it is applied in principle to construct graphs of the type shown above.
2003 - Phylogenetic analysis
You have studied the relationship between GlnRS and GluRS throughout your assignments (in 2003). An important contribution to this topic was made in September of 2003, with the discovery of a GluRS isozyme with intriguing specificity in H. pylori. It appears that GluRS-1 aminoacylates tRNAGlu while GluRS-2 specifically aminoacylates tRNAGln. Is this a missing link in the evolution of laterally transferred GlxRS ? Is it an alternative route to independent evolution of GlnRS activity ? Or is it just a refinement of the GluRS → GatABC pathway, perhaps for better metabolic regulation ? Here is some of the evidence:
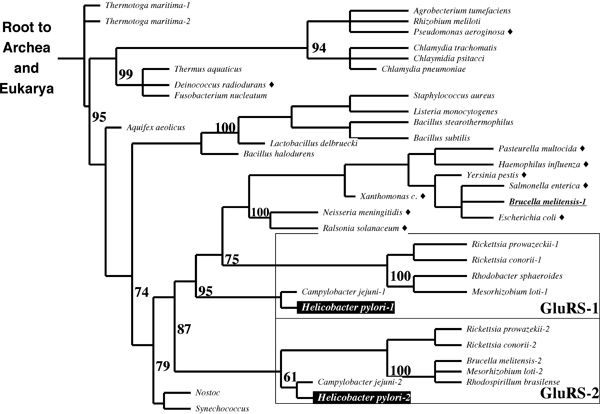
- Briefly explain the principles of this phylogenetic tree in terms of what can be observed, what must be inferred and how this tree integrates this information.
- What do the numbers in the figure mean ?
- Briefly state how the principle of maximum parsimony is applied to construct phylogenetic trees such as this one.
- Briefly discuss the evidence in the tree for (1) independent events of horizontal gene transfer into bacteria, and (2) gene duplication leading to GluRS-1 and -2.
Here are the 2004 questions to the same sketch:
- Briefly describe all input data that is required to build a phylogenetic tree like the one shown above and how the data needs to be prepared in principle.
- What do the numbers in the figure mean? How could they have they been derived? What are they useful for?
- Briefly discuss the evidence the figure shows for more than one independent event of horizontal gene transfer of GlnRS into bacteria.
2004 - Gene regulation
Here is an excerpt from a recent abstract using microarray technology to study pathogen gene regulation:
- Identification of iron-activated and -repressed Fur-dependent genes by transcriptome analysis of Neisseria meningitidis group B.
- Grifantini R, Sebastian S, Frigimelica E, Draghi M, Bartolini E, Muzzi A, Rappuoli R, Grandi G, Genco CA. Proc Natl Acad Sci U S A (2003) 100:9542-9547
- Iron is limiting in the human host, and bacterial pathogens respond to this environment by activating genes required for bacterial virulence. Transcriptional regulation in response to iron in Gram-negative bacteria is largely mediated by the ferric uptake regulator protein Fur, which in the presence of iron binds to a specific sequence in the promoter regions of genes under its control and acts as a repressor. Here we describe DNA microarray, computational and in vitro studies to define the Fur regulon in the human pathogen Neisseria meningitidis group B (strain MC58). After iron addition to an iron-depleted bacterial culture, 153 genes were up-regulated and 80 were down-regulated. [...] Forty-two promoter regions were amplified and 32 of these were shown to bind Fur by gel-shift analysis. Among these genes, many of which had never been described before to be Fur-regulated, 10 were up-regulated on iron addition, demonstrating that Fur can also act as a transcriptional activator. [...]
- Describe the principle and key steps of this experiment up to the generation of expression profiles.
- What is the "Fur regulon" ?
- After clustering genes according to the similarity of expression profiles, you obtain sets of genes with correlated expression profiles. You hypothesize that these genes may be coregulated. What bioinformatics procedure(s) can you suggest to support this idea and to identify further genes that may be regulated by the same factors?
2004 - Genomes and Pathways
Far from being dull components of dusty textbook knowledge about basic cellular metabolism, tRNA synthetases appear in surprising variations in the microbial world. Consider the excerpt from the following abstract about the very problematic human pathogen Pseudomonas aeruginosa:
- Direct glutaminyl-tRNA biosynthesis and indirect asparaginyl-tRNA biosynthesis in Pseudomonas aeruginosa PAO1.
- Akochy PM, Bernard D, Roy PH, Lapointe J. J Bacteriol. (2004) 186:767-776
- The genomic sequence of Pseudomonas aeruginosa PAO1 was searched for the presence of open reading frames (ORFs) encoding enzymes potentially involved in the formation of Gln-tRNA and of Asn-tRNA. We found ORFs similar to known glutamyl-tRNA synthetases (GluRS), glutaminyl-tRNA synthetases (GlnRS), aspartyl-tRNA synthetases (AspRS), and trimeric tRNA-dependent amidotransferases (AdT) but none similar to known asparaginyl-tRNA synthetases (AsnRS).
- [ The authors then describe biochemical experiments to conclude ... ]
- [ The authors then describe biochemical experiments to conclude ... ]
- These results show that P. aeruginosa PAO1 is the first organism known to synthesize Asn-tRNA via the indirect pathway and to synthesize Gln-tRNA via the direct pathway. The essential role of AdT in the formation of Asn-tRNA in P. aeruginosa and the absence of a similar activity in the cytoplasm of eukaryotic cells identifies AdT as a potential target for antibiotics to be designed against this human pathogen. Such novel antibiotics could be active against other multidrug-resistant gram-negative pathogens such as Burkholderia and Neisseria as well as all pathogenic gram-positive bacteria.
- Briefly describe the computational strategy the authors probably used and what data/resources were required.
- Discuss briefly how you could expand this procedure, to systematically identify many more potential P. aeruginosa drug targets. (To answer this, you will need to think a little about what makes a promising drug target ...)
2004 - Protein Structure Domains
The recognition that proteins are frequently organized into domains and that domains appear to provide an efficient means of combinatorial generation of function, is one of the remarkable insights we have gained from structural studies and sequence analysis. Among the numerous different definitions for "protein domains" are the following:
A domain is:
- an independently folding fragment of protein sequence
- a separately inherited sequence fragment
- a compact set of amino acids in a structure
- a functional unit
- Briefly sketch a possible computational strategy for two of these definitions in order to use data from primary(!) databases to compile a list of the domains they contain.
You could structure this as follows:
- Propose a computable procedure that relates to one of the definitions
- Define the source data it would operate on
- Describe how the source data would be used in a bioinformatics procedure
- Note what results this would yield and how the result would relate to the definition
2004 - Homology modeling and model analysis
We have studied protein structure and homology models in the assignments and discussed them at length in various lectures. The single most important factor affecting the quality of a homology model is the sequence alignment to the template and this can be a problem, since optimal sequence alignment and "true" structure alignment need not always coincide. However, there are also other factors to be considered, especially when you have more than one homologuos structure that could serve as a template.
Short answers please!
- Discuss briefly how the overall degree of target–template sequence similarity would affect your choice of a template structure for homology modeling if you had several options available. Would you rather choose a closely related paralogue or a more distantly related orthologue?
- Discuss briefly how the presence of insertions or deletions in target–template alignments would affect your choice of a template structure for homology modeling if you had several options available.
- The Thermus thermophilus GluRS structure with the PDB-ID 1N78 contains the substrate analogon glutamol-AMP. Can you copy and paste coordinates for glutamate and ATP from the structure file 1J09 (Thermus thermophilus GluRS structure solved at 1.9Å resolution and containing both glutamate and ATP.) into a homology model based on 1N78? If yes, how? If no, why not?
- You have commented in several parts of your assignments on the presence of hydrogen bonds. Please sketch an approximate distribution of N – O distances in protein structure hydrogen bonds, pointing out minimum, maximum and mode of the distribution.
- The following two records have been copied from the coordinate file 1N78.PDB. From the information given here, explain what these atoms appear to be a part of, what relationship they could have to each other and how one could calculate the distance between the atoms (in Å)?
[...] ATOM 66 OG SER A 9 42.867 81.416 54.733 1.00 28.69 O [...] ATOM 9214 O3* A C 576 41.539 83.821 54.257 1.00 73.54 O [...]
2005 - Genome Analysis
Monodelphis Domestica - the short-tailed opposum, a marsupial - is one of the organisms whose genome has recently been sequenced and made available on the Ensembl Website. This section discusses some issues in assembling the genome, defining the genes and arriving at annotations. From the Ensembl Web site:
|
- Briefly decribe how the genome has been sequenced and assembled.
From the Ensembl Web site:
- "The gene set for Opossum was built using a modified version of the standard Ensembl genebuild pipeline. The species-specific sequence resources (opossum cDNA and protein) are very limited, so the vast majority of gene models are based on genewise alignments of proteins from other species. Most of the proteins being aligned were from species genetically distant to opossum. To improve the accuracy of models generated from these proteins, the genewise alignments were made to stretches of genomic sequence[...]. Opossum and human cDNAs were aligned and used to add UTRs to the genewise predictions where possible. The gene models were assessed by generating sets of potential orthologs to genes from other mammalian species. [...]"
- What is a gene model?
BLASTing the Ets-1 transcription factor winged helix domain sequence from 1DUX.PDB against the M. Domestica scaffolds (TBLASTN) yields a highly conserved match:
Query Scaffold Stats Start End Ori Name Start End Ori Score E-val %ID Length 1 70 + scaffold_134 566740 566946 + 551 1.4e-58 91.43 70
This match has a link to the ensembl genome browser, leading to the following screen (slightly edited):
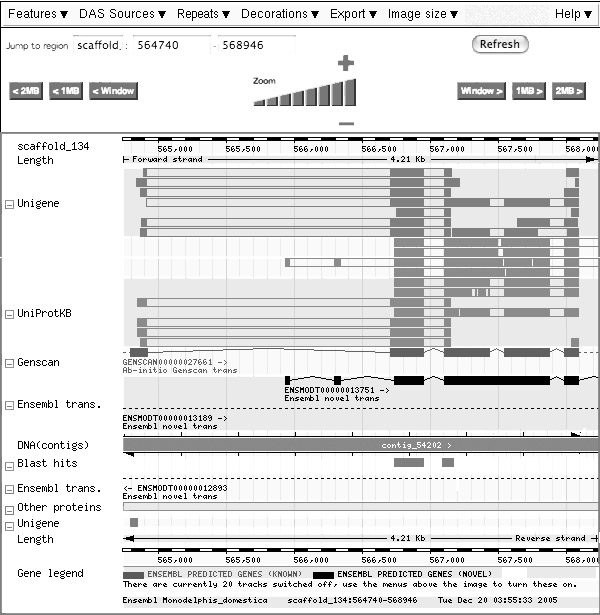
A feature in the sense used here is an annotation that is attached to a position of the genomic sequence.
- Identify the feature that shows where the region of sequence similarity has been found.
- Identify the feature that corresponds to the current gene model which the similarity search has retrieved.
- Identify the feature that corresponds to the observed protein sequence which best supports the current gene model.
- Identify the features that correspond to nucleotide sequences of similar proteins.
- Is the current gene model consistent with all observations integrated here? Explain.
This question intentionally focussed on a site that the class had (most likely) not encountered so far, in order to evaluate the transfer of knowledge into a new context. Tools on the Web change rapidly.
2005 - Model and Algorithm
In the post-genomic era, many of us perceive the comprehensive enumeration of all protein-protein interactions to be the logical and necessary next step, following after genome analysis. Obviously, careful experimental analysis does not scale well for a problem of this magnitude (a chordate organism may have on the order of half a million protein-protein interactions, not even counting small molecules, DNA and RNA) and for the foreseeable future computational procedures are going to play a crucial role in this endeavor. After all, experimental procedures can only determine the physical aspect of interactions; hoewever, whether an interaction is important for the organism, i.e. whether it contributes to the organism's fitness function - the interaction is conserved - is a question from the domain of theoretical analysis.
In a recent seminar, Elisabeth Tillier discussed computational methods for the prediction of protein-protein interactions. While her own approach is based on the analysis of co-evolutionary change in protein pairs, four other computational methods were briefly mentioned. These are the analysis of:
- Conservation of gene order;
- Gene fusion events;
- Correlated expression profiles; and
- Phylogenetic profiles.
- For two of these methods describe briefly (short answers, keywords):
- the underlying biology that gives rise to the observation;
- the input data that is required;
- the computational process;
- the types of results that can be expected and how they would be interpreted;
- if the result is a yes/no answer: how the process' performance can be evaluated;
- if the process results in a probability for interaction: how a decision-threshold can be determined
2006 Data, Concept and Procedure
STRING at the EBI is a search tool for known and predicted functional protein/protein interactions. STRING quantitatively integrates interaction data for a large number of organisms, and transfers information between these organisms where applicable. Obviously, experimental results are included in the prediction, but there are also a number of purely theoretical approaches:
The following theoretical approaches contribute to the prediction:
- Gene neighborhood: in prokaryotes, functionally related genes are often found in operons.
- Gene fusion: interacting proteins are sometimes found to be fused in related organisms.
- Gene occurrence (Phylogenetic profile): the conservation of a gene across a large number of species can be summarized in a profile that characterises the distribution of this gene accross evolution. Functionally related genes often have similar profiles.
- Gene sets in databases: Curated datasets such as MIPS complexes or KEGG pathways contain sets of genes that form known functional units.
- For two of these approaches:
- define the source data that is being used,
- describe the rationale behind applying the biological observation to predict a physical or functional interaction,
- outline the computational procedure for the interaction prediction, and
- explain how to interpret the results.
- For one of the two approaches you have described: how would you evaluate the significance of the prediction?
2006 Computational Systems Biology
One of the objectives of "post-genomic" science is to define the systems that operate inside a cell and identify the genes and gene products that are associated with them. A top-down approach could start from a particular phenotype or physiological capacity and define from first principles the systems that would be required to generate such behaviour, then one would search for molecular components that would match the postulated systems. A bottom-up approach could look at high-resolution cross-sectional datasets, such as expression profiles, interaction maps, phylogenetic footprints etc., use clustering tools to identify systems of collaborating genes and then ask which role we could ascribe to these systems.
Here is an example of typical hybrid bioinformatics / experimental approach
- Computational discovery of gene modules and regulatory networks
Bar-Joseph Z, Gerber GK, Lee TI, Rinaldi NJ, Yoo JY, Robert F, Gordon DB, Fraenkel E, Jaakkola TS, Young RA, Gifford DK.
Nat Biotechnol. 2003 Nov;21(11):1337-1342
- We describe an algorithm for discovering regulatory networks of gene modules, GRAM (Genetic Regulatory Modules), that combines information from genome-wide location and expression data sets. A gene module is defined as a set of coexpressed genes to which the same set of transcription factors binds. Unlike previous approaches that relied primarily on functional information from expression data, the GRAM algorithm explicitly links genes to the factors that regulate them by incorporating DNA binding data, which provide direct physical evidence of regulatory interactions. We use the GRAM algorithm to describe a genome-wide regulatory network in Saccharomyces cerevisiae using binding information for 106 transcription factors profiled in rich medium conditions with data from over 500 expression experiments. We also present a genome-wide location analysis data set for regulators in yeast cells treated with rapamycin, and use the GRAM algorithm to provide biological insights into this regulatory network
Essentially, this strategy combines ChIP-Chip(*) data and expression profiles. These two methods have orthogonal weaknesses: TF location analysis does not distinguish activation from repression; similar expression profiles may be observed from genes that are under the control of different regulatory systems.
(*)ChIP-chip: Chromatin Immunoprecipitation on chips: Transcription factors (TF), bound to their cognate sequence, are crosslinked with chromosomal DNA. The DNA is fragmented into ~500 nt pieces. Crosslinked TF-DNA complexes are immunoprecipitated with antiTF antibodies. Subsequently the cross-links are broken and protein removed by enzymatic digestion. The DNA fragments are then labelled with a fluorophore and identified on a microarray chip.
- Briefly sketch the steps in the autor's procedure to combines a ChIP-chip data set with a set of clusters of expression profiles, in order to validate which of the genes that are members of a cluster are candidates to be a component of a "gene module".
- What is the underlying hypothesis that makes such a refinement approach reasonable?
- Briefly explain how GO annotations could be used to further refine gene module membership.
All the information that is needed is actually given in the question, this question relates solely to a structured approach to define computational and/or experimental data, understand how the data reflects a particular biological phenomenon and integrate the data.
2010 Phylogenetic analysis
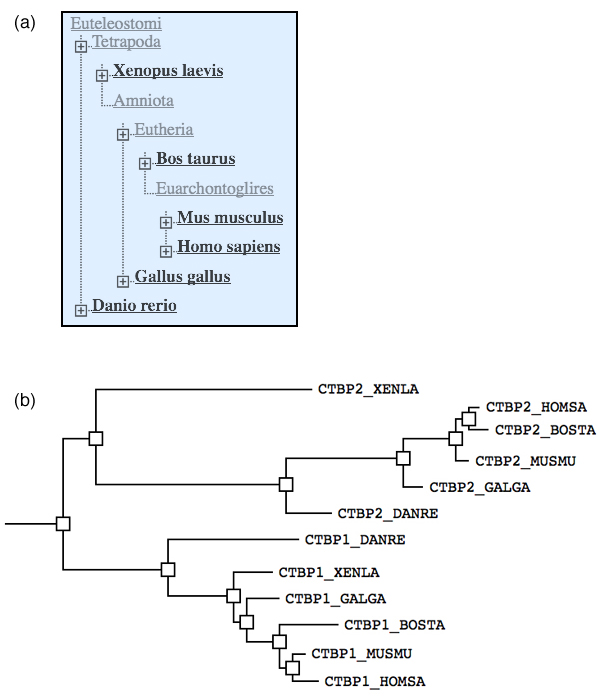
- How can you distinguish between speciation and duplication events in such a tree?
- Label the speciation and duplication branchpoint(s) in this tree with "D" / "S".
- How many CTBP homologs did the Euteleostomi cenancestor have?
To answer the last question, you have to understand which branch(es) in the tree belong to the first species that were descended from the cenancestor.