Lecture 01
Update Warning! This page has not been revised yet for the 2007 Fall term. Some of the slides may be reused, but please consider the page as a whole out of date as long as this warning appears here.
Organisation and Orientation
Add/expand:
- Mission: Analysis must be followed by interpretation, course is hands-on, interactive and goal oriented.
- Technology: Google group, Wiki, supporting material on the Web
- Contact information
- Technical details of course organisation
Lecture Slides
Slide 001
Slide 002
Slide 003
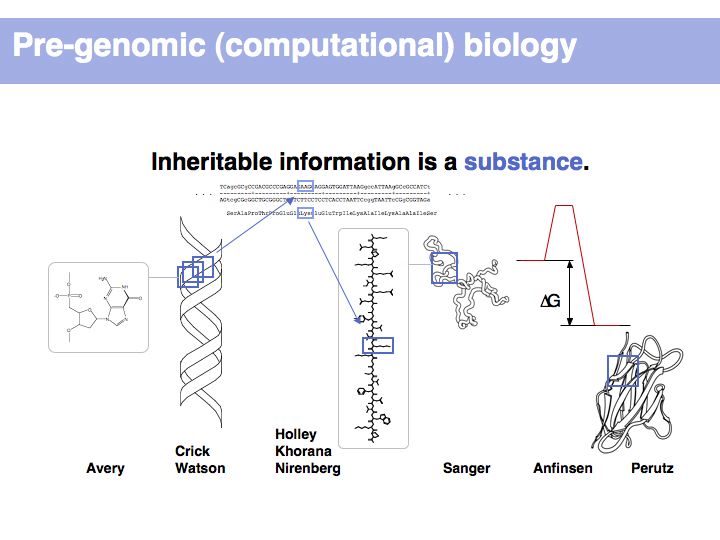
From its beginning, it was recognized that molecular biology is an information science, just as much as a molecular science. The abstractions and models that focus on the essence of this information, rather than on the details of its representation, have proven to be remarkably powerful in explaining the basic features of life, such as inheritance, self-organization and the process of evolution.
Slide 004
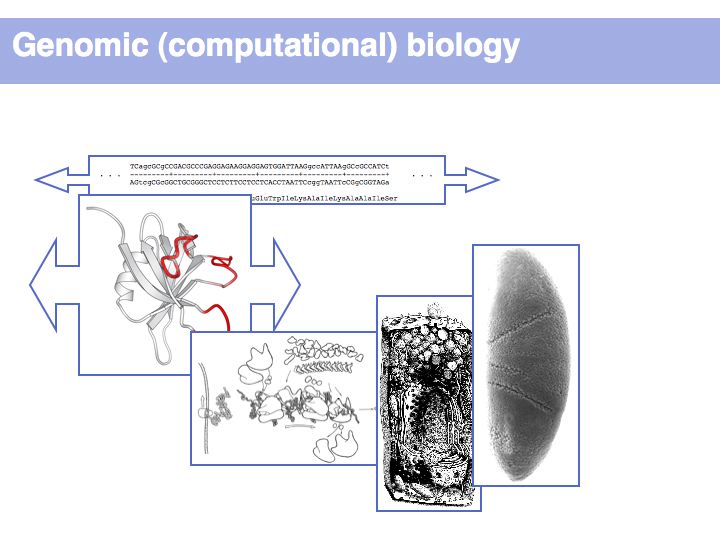
The promises of genome analysis include harnessing the power of self assembly towards a bio-nanotechnologic revolution: growth, rather than manufacturing. This includes the vision of regenerative molecular medicine, essentially relegating disease to the dark past ages of ignorance. But while the information for a complete specification of life is undoubtedly present in the genome, life realizes itself in complex interactions between context-dependent components. This makes life essentially unpredictable, at least to our current approaches. The sheer volume of data is a comparatively minor obstacle.
Slide 005
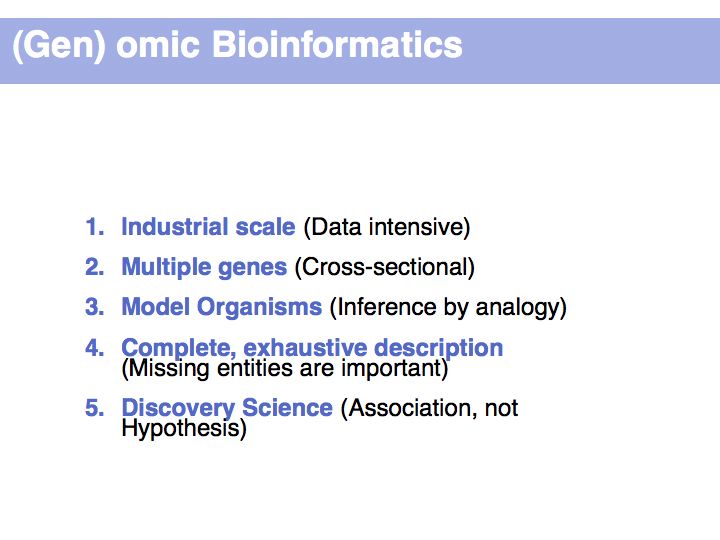
The current emphasis on -omic sciences creates novel challenges both in the quantity as well as the quality of scientific enquiry. The scale has become larger; molecular components are analyzed not in isolation but in their associations;comparison between genes within and across species is a major source of new insight and the absence of particular components and features is just as informative as their presence. However the availability technology should not lead to a purely methods-driven agenda.
Slide 006
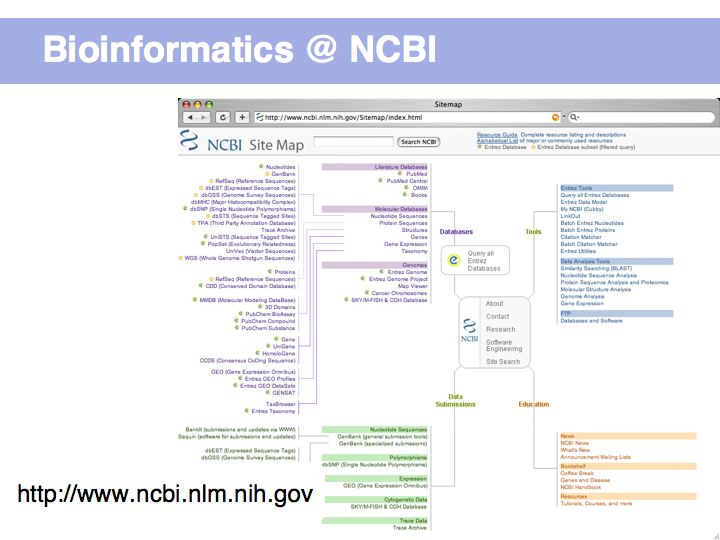
The US National Center of Biotechnology Information is one of the world's major centres for molecular data.
Slide 007
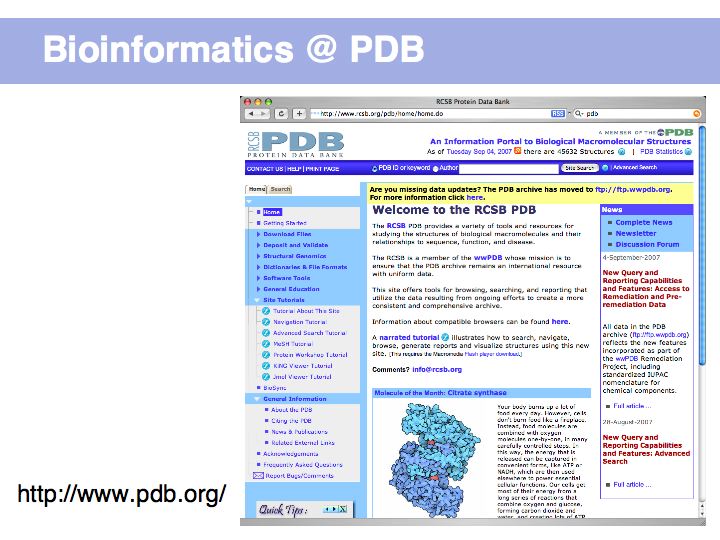
The PDB (Protein structure DataBase) is the world's central repository for 3D structural data of proteins and nucleic acids.
Slide 008
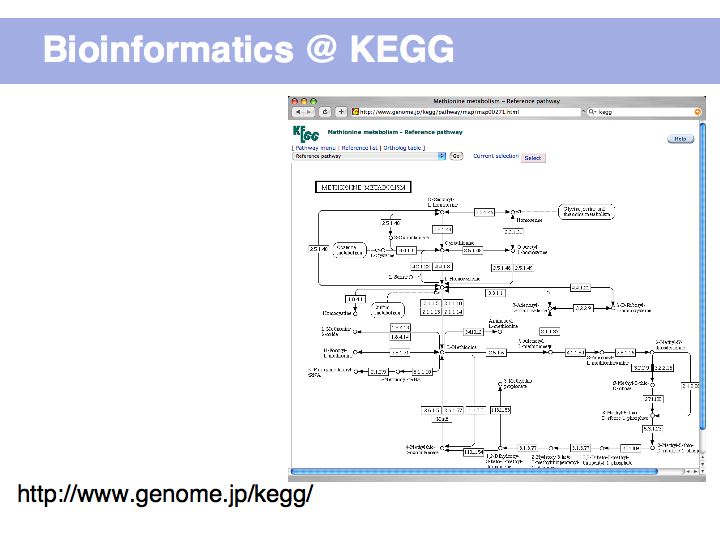
KEGG (the Kyoto Encyclopedia of Genes and Genomes) is one of a group of data resources that focus on the functional relationships of the components of biological systems. Note that sequences, structures and functions are complementary aspects of the same molecular entities. Cross-referencing between databases and ensuring consistency is a major challenge and task of biological datat management.
Slide 009

On one hand, we can conclude that biological data management is what bioinformatics is all about. On the other hand, bioinformatics as a science is a way to study biology. And this aspect - which I like to refer to as "Computational Biology" - is not well described by data management. It has a lot more to do with modeling, and the question of understanding biology.
Slide 010
Slide 011
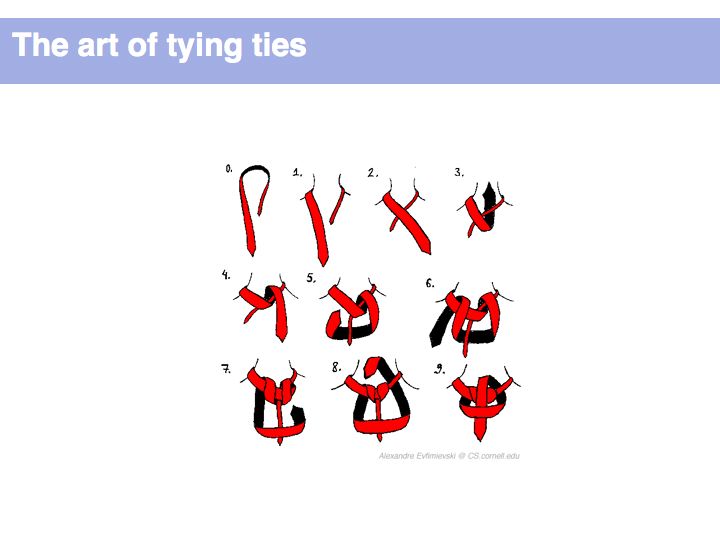
Tying ties may be at first an intimidatingly imprecise task, and indeed irrelevant. (Half of North Americans are not eligible to wear a tie, even to formal occasions, and those of the other half who are not working in a bank will maybe wear a tie on only two occasions and have the tie tied for them on the second one. Tying ties is, alas, a cultural technique that appears to be on the decline.). But it is a nice example for abstracting a complicated process down to its essential principles, and reasoning formally about these principles to obtain rigorous results about the process.
Here is an example of a systematic, albeit informal description of the process of how to tie a tie. But why is the process divided into exactly these steps? Are all of them necessary? How do we describe this process so that we can remember it ? Or do we need to refer to the sequence of images every time we would like to tie this knot? Is this a simple, or a rather complicated way to tie a tie; are there others? Are there better ways to tie a tie, and what could better even mean?
Slide 012
Slide 013
Slide 014
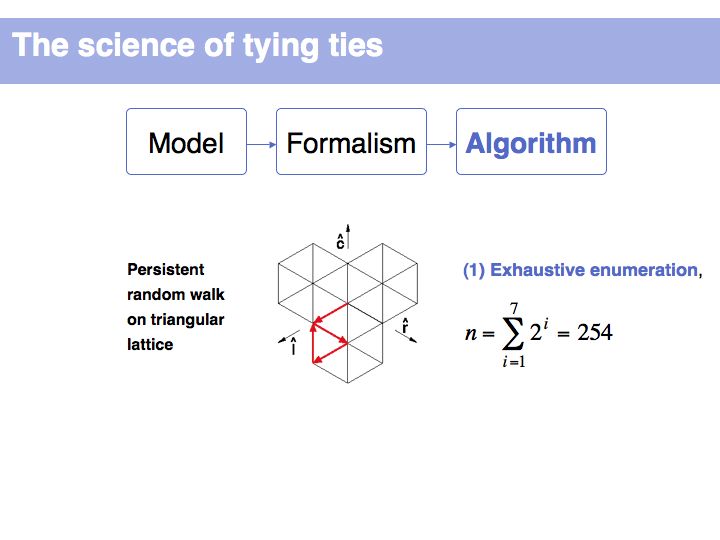
How many alternatives do we have to consider, if we allow maximally nine moves and require at least three for the finishing moves? We noted previously that there are two possibilities for the three finishing moves (LRC or RLC). Since moves cannot repeat into the same sector, each of the possibilities can only have been preceded by two alternatives i.e.. (CLRC or RLRC) and (CRLC or LRLC). These four possibilities again can have been preceded by two alternatives each ... etc. Since we treat knots of different move-numbers as distinct, the total number of moves up to length L is the sum of all powers of 2 up to (L-2). (-2 because of the finishing moves!). You should be able to figure out reasoning like this on your own!
Slide 015
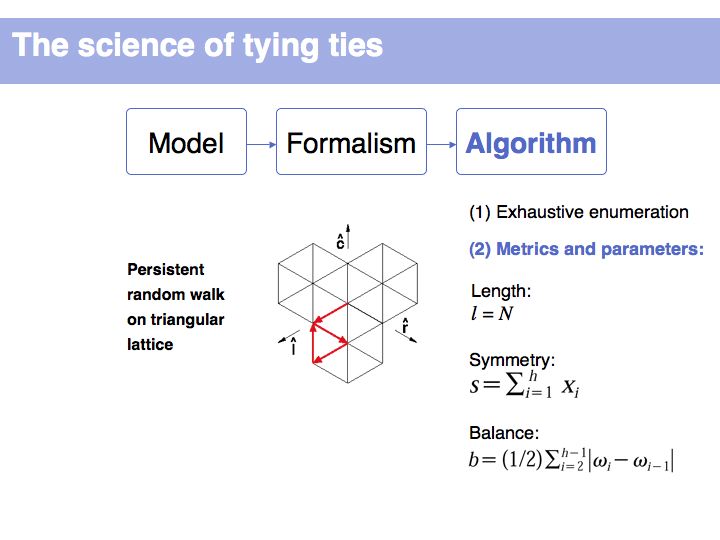
It is not uncommon for models to be bounded by constraints. Here we define metrics for symmetry and balance and we can then use parameters to judge whether certain of the 254 possible walks are acceptable or not. (Details omitted, refer to the original paper of Fink and Mao, 1999, Nature, 398:31-32 (pdf)).
Slide 016
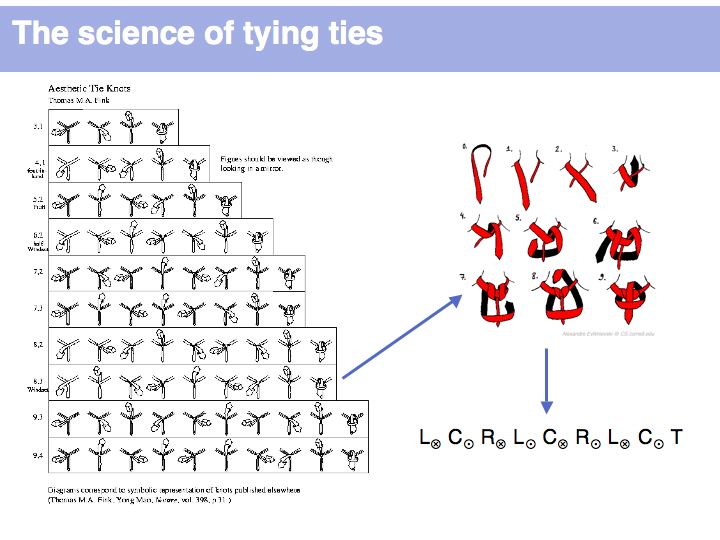
The algorithm to creat all knots, finally, requires no more than exhaustive enumeration. What is important is to note the word "exhaustive". The result is complete, in the sense that every way to tie a tie has been captured. What is not in this list, cannot exist (under the assumptions the models makes). If this reminds you of the concept of "complete information" in the sequence of a genome, this is intended.
Slide 017
Slide 018
Slide 019
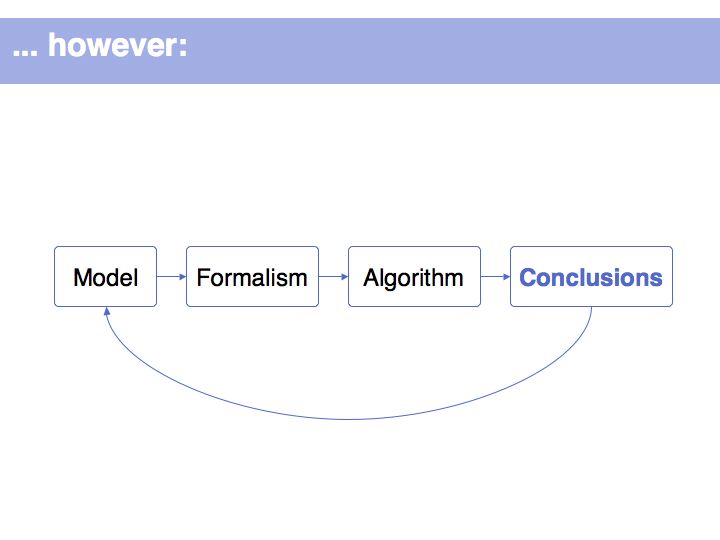
While data technologies' goals and endpoints are obvious and straightforward to define, the same does not hold true for the modeling aspect of bioinformatics. Models cannot be derived directly from an observation of the data! They require insight, judgment and a sense of perspective and direction. If the modeling exercise does not lead to valuable and testable conclusions, it is pointless.
Slide 020
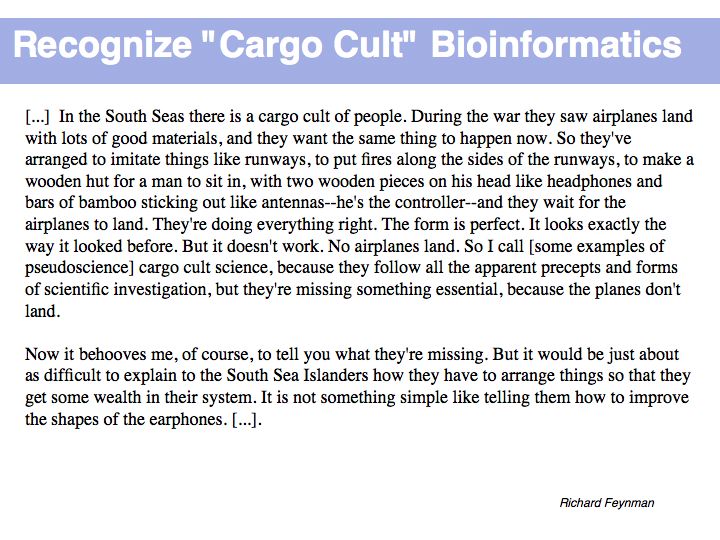
From Feynman's 1974 Caltech commencement address (e.g. see Cargo Cult Science on Wikipedia).
But what are the airplanes of bioinformatics in the first place? And how do we construct strategies of scientific enquiry that not only include the airplanes but that also make them land?
Slide 021
Slide 022
Slide 023
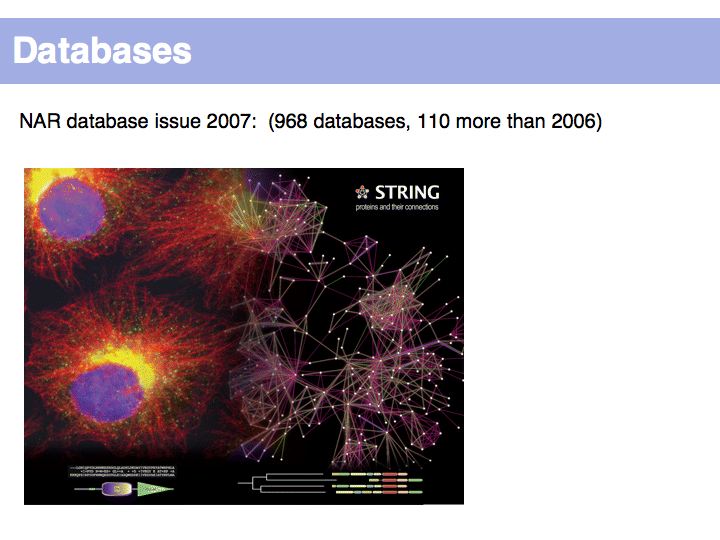
Taken from the 2007 special issue on databases, published by NAR.
By the time it takes you to study the existing databases, the majority will have seen significant updates and upgrades, gone out of existence or been superseded by more appropriate resources.