Difference between revisions of "BIO Assignment Week 4"
Line 380: | Line 380: | ||
} | } | ||
ids <- getDBid(database, table= "protein", ...) | ids <- getDBid(database, table= "protein", ...) | ||
− | seq <- db$protein[ids, "sequence"] | + | seq <- db$protein[db$protein$id == ids, "sequence"] |
return(toupper(seq)) | return(toupper(seq)) | ||
} | } |
Revision as of 14:36, 12 October 2015
Assignment for Week 4
Sequence alignment
< Assignment 3 | Assignment 5 > |
Note! This assignment is currently active. All significant changes will be announced on the mailing list.
Concepts and activities (and reading, if applicable) for this assignment will be topics on next week's quiz.
Contents
- Take care of things, and they will take care of you.
- Shunryu Suzuki
Introduction
Sequence alignment is a very large, and important topic.
One of the foundations of bioinformatics is the empirical observation that related sequences conserve structure, and often function. Much of what we know about a protein's physiological function is based on the conservation of that function as the species evolves. We assess conservation by comparing sequences between related proteins. Conservation - or its opposite: variation - is a consequence of selection under constraints: protein sequences change as a consequence of DNA mutations, this changes the protein's structure, this in turn changes functions and that has multiple effects on a species' reproductive fitness. Detrimental variants may be removed. Variation that is tolerated is largely neutral and therefore found only in positions that are neither structurally nor functionally critical. Conservation patterns can thus provide evidence for many different questions: structural conservation among proteins with similar 3D-structures, functional conservation among homologues with comparable roles, or amino acid propensities as predictors for protein engineering and design tasks.
This is the basis on which we can make inferences from well-studied model organisms in species that have not been studied as deeply. And the foundation of discovering relatedness is to measure protein sequence similarity. If two sequences are much more similar than we could expect from chance, we hypothesize that their similarity comes from shared ancestry. The measurement of sequence similarity however requires sequence alignment[1].
Therefore a carefully done sequence alignment is a cornerstone for the annotation of the essential properties a gene or protein. It can already tell us a lot about which proteins we expect to have similar functions in different species.
Multiple sequence alignments (MSAs) are further useful to resolve ambiguities in the precise placement of indels and to ensure that columns in alignments actually contain amino acids that evolve in a similar context. MSAs serve as input for
- functional annotation;
- protein homology modelling;
- phylogenetic analyses, and
- sensitive homology searches in databases.
In this assignment we will explore the essentials of
- optimal global and local alignment;
- BLAST searches for best matches;
- PSI BLAST searches for exhaustive matches; and
- Multiple sequence alignments.
As usual, the focus will be on practical, hands on approaches.
This is the scenario: you have previously identified a best match for a Mbp1 relative in YFO. Is this the most closely related protein? Is its DNA binding domain conserved? How can we identify all related genes in YFO? And, what can we learn from such a collection?
Optimal sequence alignments
Let's start by aligning the sequences of Mbp1 and the YFO relative. For simplicity, I will call the two proteins MBP1_SACCE
and MBP1_YFORG
through the remainder of the assignment, and even if I casually refer to a gene when I'm really talking about a protein (sorry), you should recognize from context what is meant.
Preparation: Updated Database Functions
First we need to pull out the two sequences from the database object we created last time. You could recreate the state of your database by re-running the relevant parts of the script, or piece things together from the code of the previous assignment.
Keeping things in scripts is really useful.
But since we'll be working more with our database, adding to the data model, updating code for getting and setting data, and adding proteins, annotations and cross-references, let's spend a moment to organize things in a more principled way.
- We should create a script that loads the functions to manage the database;
- We should save our database so we can easily reload the contents.
Task:
Here's how we should organize this:
- We'll define a variable called
PROJECTDIR
which automatically gets set whenever you startup R. - A scriptfile with the necessary functions should automatically get
source()
'd at startup; - The database should be saved so it can easily be loaded.
You will find the code below. It looks long, but it's really quite straightforward bookkeeping. I have added a number of tests to help make sure the input is sane. That actually makes up the majority of the code. Sanitizing user input is always much more effort than the actual algorithm. I have tested the functions and think they should work as expected. But if you come across a situation where your input produces an error, or creates an inconsistency in the database, by all means let me know so the code can be improved.
1. Create a project directory for the assignments on your computer if you don't have one yet.
2. Adapt the code below as needed, and execute it to update .Rprofile
.
file.edit("~/.Rprofile")
# Add:
PROJECTDIR <- "full/path/to/your/directory/" # including the final backslash.
source(paste(PROJECTDIR, "dbUtilities.R", sep=""))
# ... and save the file.
# To make the definition available, run it.
source("~/.Rprofile")
# Now let's create the script for the database functions:
file.edit(paste(PROJECTDIR, "dbUtilities.R", sep=""))
An edit window for the file has opened. Copy the entire code block below, and paste it into the editor.
# dbUtilities.R
#
# Purpose: Utility functions for a Protein datamodel
# Version: 0.1
# Date: Oct 2015
# Author: Boris and class
#
# ToDo: Add more tables.
# Accept either taxonomy_id OR species name
# and pull the other from NCBI.
# Notes: Cf. schema sketch at
# http://steipe.biochemistry.utoronto.ca/abc/index.php/File:ProteinDataModel.1.jpg
# Currently implements only "protein" and
# "taxonomy" table.
# ==========================================================
# ==== FUNCTIONS =========================================
# ==== createDB =============================================
# Returns an empty list
# We use a separate function because we might want to
# some initialization code later.
createDB <- function() {
return(list())
}
# ==== in2seq ==============================================
# Utility function to sanitize input and convert it into a
# sequence string. Case can be optionally changed.
# Letters that are not one-letter code - such as
# ambiguity codes - throw an error if not explicitly
# permitted.
in2seq <- function(s, uc = FALSE, lc = FALSE, noAmbig = TRUE) {
s <- paste(unlist(s), collapse="") # flatten whatever structure it has
s <- gsub("[^a-zA-Z]", "", s)
if (noAmbig) {
ambCodes <- "([bjouxzBJOUXZ])" # parentheses capture the match
ambChar <- unlist(regmatches(s, regexec(ambCodes, s)))[1]
if (! is.na(ambChar)) {
stop(paste("Input contains ambiguous letter: \"", ambChar, "\"", sep=""))
}
}
if (uc) { s <- toupper(s)}
if (lc) { s <- tolower(s)}
return(s)
}
# ==== in2vec ==============================================
# Utility function to sanitize input and expand it into a
# vector of single characters. Arguments for in2seq are
# passed through via the three-dots parameter syntax.
in2vec <- function(s, ...) {
s <- in2seq(s, ...)
return(unlist(strsplit(s, "")))
}
# ==== addToDB =============================================
# Add a new protein entry to the database, with associated
# taxonomy entry
addToDB <- function(database,
name = "",
refseq_id = "",
uniprot_id = "",
taxonomy_id,
genome_xref = numeric(),
genome_from = numeric(),
genome_to = numeric(),
sequence = "",
species_name = "") {
if (missing(database)) {
stop("\"database\" argument is missing with no default.")
}
if (missing(taxonomy_id)) {
stop("taxonomy_id argument is missing with no default.")
}
if (! is.numeric(taxonomy_id)) {
stop(paste("taxonomy_id \"",
taxonomy_id,
"\" is not numeric. Please correct.", sep=""))
}
# check taxonomy_id
if (! any(database$taxonomy$id == taxonomy_id)) { # new taxonomy_id
if (missing(species_name)) {
stop(paste("taxonomy_id",
taxonomy_id,
"is not yet in database, but species_name",
"is missing with no default."))
}
else {
# add this species to the taxonomy table
database$taxonomy <- rbind(database$taxonomy,
data.frame(id = taxonomy_id,
species_name = species_name,
stringsAsFactors = FALSE))
}
}
# handle protein
# pid is 1 if the table is empty, max() + 1 otherwise.
if (is.null(nrow(database$protein))) { pid <- 1 }
else {pid <- max(database$protein$id) + 1}
database$protein <- rbind(database$protein,
data.frame(id = pid,
name = name,
refseq_id = refseq_id,
uniprot_id = uniprot_id,
taxonomy_id = taxonomy_id,
genome_xref = genome_xref,
genome_from = genome_from,
genome_to = genome_to,
sequence = in2seq(sequence),
stringsAsFactors = FALSE))
return(database)
}
# ==== setDB ===============================================
# Update database values
setDB <- function(database,
table,
id = NULL,
name = NULL,
refseq_id = NULL,
uniprot_id = NULL,
taxonomy_id = NULL,
genome_xref = NULL,
genome_from = NULL,
genome_to = NULL,
sequence = NULL,
species_name = NULL) {
if (missing(database) | missing(table)) {
stop("Database or table is missing with no default.")
}
if (table == "protein") {
if (is.null(id)) {
stop("Protein id is missing with no default.")
}
row <- which(database$protein$id == id)
if (! is.null(name)) { database$protein[row, "name"] <- as.character(name) }
if (! is.null(refseq_id)) { database$protein[row, "refseq_id"] <- as.character(refseq_id) }
if (! is.null(uniprot_id)) { database$protein[row, "uniprot_id"] <- as.character(uniprot_id) }
if (! is.null(taxonomy_id)) {
# must be numeric ...
if (! is.numeric(taxonomy_id)) {
stop(paste("taxonomy_id",
taxonomy_id,
"is not numeric. Please correct."))
}
# must exist in taxonomy table ...
if (! any(database$taxonomy$id == taxonomy_id)) { # new taxonomy_id
stop(paste("taxonomy_id",
taxonomy_id,
"not found in taxonomy table. Please update taxonomy table and try again."))
}
# all good, update it...
database$protein[row, "taxonomy_id"] <- taxonomy_id
}
if (! is.null(genome_xref)) { database$protein[row, "genome_xref"] <- genome_xref}
if (! is.null(genome_from)) { database$protein[row, "genome_from"] <- genome_from}
if (! is.null(genome_to)) { database$protein[row, "genome_to"] <- genome_to}
if (! is.null(sequence)) { database$protein[row, "sequence"] <- in2seq(sequence)}
}
else if (table == "taxonomy") {
if (missing(taxonomy_id)) {
stop("taxonomy_id is missing with no default.")
}
if (! any(database$taxonomy$id == taxonomy_id)) {
stop(paste(" Can't set values for this taxonomy_id.",
taxonomy_id,
"was not found in taxonomy table."))
}
row <- which(database$taxonomy$id == taxonomy_id)
if (species_name != "") { database$taxonomy[row, "species_name"] <- species_name }
}
else {
stop(paste("This function has no code to update table \"",
table,
"\". Please enter a valid table name."))
}
return(database)
}
# ==== getDBid =============================================
# Get a vector of IDs from a database table from all rows
# for which all of the requested attributes are true.
# Note: if no restrictions are entered, ALL ids are returned.
# We don't have code to select from genome coordinates, or
# query from sequence.
getDBid <- function(database,
table,
name = NULL,
refseq_id = NULL,
uniprot_id = NULL,
taxonomy_id = NULL,
species_name = NULL) {
if (missing(database) | missing(table)) {
stop("Database or table is missing with no default.")
}
if (table == "protein") {
sel <- rep(TRUE, nrow(database$protein)) # initialize
if (! is.null(name) ) { sel <- sel & database$protein[, "name"] == name }
if (! is.null(refseq_id) ) { sel <- sel & database$protein[, "refseq_id"] == refseq_id }
if (! is.null(uniprot_id) ) { sel <- sel & database$protein[, "uniprot_id"] == uniprot_id }
if (! is.null(taxonomy_id)) { sel <- sel & database$protein[, "taxonomy_id"] == taxonomy_id }
sel <- db$protein$id[sel] # get ids by selecting from vector
}
else if (table == "taxonomy") {
sel <- rep(TRUE, nrow(database$taxonomy)) # initialize
if (! is.null(taxonomy_id) ) { sel <- sel & database$taxonomy[, "id"] == taxonomy_id }
if (! is.null(species_name)) { sel <- sel & database$taxonomy[, "species_name"] == species_name }
sel <- db$taxonomy$id[sel] # get ids by selecting from vector
}
else {
stop(paste("This function has no code to select from table \"",
table,
"\". Please enter a valid table name."))
}
return(sel)
}
# ==== getSeq ==============================================
# Retrieve the sequences for given id matches from the
# protein table. Uppercase, to make Biostrings happy.
getSeq <- function(database, ...) {
if (missing(database)) {
stop("Database argument is missing with no default.")
}
ids <- getDBid(database, table= "protein", ...)
seq <- db$protein[db$protein$id == ids, "sequence"]
return(toupper(seq))
}
# ==== MESSAGE ============================================
cat("db_utilities.R has been loaded. The following functions are now available:\n")
cat(" createDB()\n")
cat(" addToDB()\n")
cat(" setDB()\n")
cat(" getDBid()\n")
cat(" getSeq()\n")
cat(" in2seq()\n")
cat(" in2vec()\n")
cat(" \n")
# ==== TESTS =============================================
# TBD
# [END]
Save dbUtilities.R and source()
it to make the functions immediately available. They will also be available when you next start R.
source(paste(PROJECTDIR, "dbUtilities.R", sep=""))
We now have a first set of somewhat credible database functions. Let's create a database and add two proteins.
db <- createDB()
db <- addToDB(db,
name = "Mbp1",
refseq_id = "NP_010227",
uniprot_id = "P39678",
taxonomy_id = 4932,
genome_xref = "NC_001136.10",
genome_from = 352877,
genome_to = 355378,
sequence = "
1 msnqiysary sgvdvyefih stgsimkrkk ddwvnathil kaanfakakr trilekevlk
61 ethekvqggf gkyqgtwvpl niakqlaekf svydqlkplf dftqtdgsas pppapkhhha
121 skvdrkkair sastsaimet krnnkkaeen qfqsskilgn ptaaprkrgr pvgstrgsrr
181 klgvnlqrsq sdmgfprpai pnssisttql psirstmgpq sptlgileee rhdsrqqqpq
241 qnnsaqfkei dledglssdv epsqqlqqvf nqntgfvpqq qssliqtqqt esmatsvsss
301 pslptspgdf adsnpfeerf pgggtspiis miprypvtsr pqtsdindkv nkylsklvdy
361 fisnemksnk slpqvllhpp phsapyidap idpelhtafh wacsmgnlpi aealyeagts
421 irstnsqgqt plmrsslfhn sytrrtfpri fqllhetvfd idsqsqtvih hivkrksttp
481 savyyldvvl skikdfspqy rielllntqd kngdtalhia skngdvvffn tlvkmgaltt
541 isnkegltan eimnqqyeqm miqngtnqhv nssntdlnih vntnnietkn dvnsmvimsp
601 vspsdyityp sqiatnisrn ipnvvnsmkq masiyndlhe qhdneikslq ktlksisktk
661 iqvslktlev lkesskdeng eaqtnddfei lsrlqeqntk klrkrliryk rlikqkleyr
721 qtvllnklie detqattnnt vekdnntler lelaqeltml qlqrknklss lvkkfednak
781 ihkyrriire gtemnieevd ssldvilqtl iannnknkga eqiitisnan sha
",
species_name = "Saccharomyces cerevisiae")
db <- addToDB(db,
name = "Res2",
refseq_id = "NP_593032",
uniprot_id = "P41412",
taxonomy_id = 4896,
genome_xref = "NC_003424.3",
genome_from = 686543,
genome_to = 689179,
sequence = "
1 maprssavhv avysgvevye cfikgvsvmr rrrdswlnat qilkvadfdk pqrtrvlerq
61 vqigahekvq ggygkyqgtw vpfqrgvdla tkykvdgims pilsldideg kaiapkkkqt
121 kqkkpsvrgr rgrkpsslss stlhsvnekq pnssisptie ssmnkvnlpg aeeqvsatpl
181 paspnallsp ndntikpvee lgmleapldk yeeslldffl hpeegripsf lyspppdfqv
241 nsvidddght slhwacsmgh iemiklllra nadigvcnrl sqtplmrsvi ftnnydcqtf
301 gqvlellqst iyavdtngqs ifhhivqsts tpskvaaaky yldcilekli siqpfenvvr
361 lvnlqdsngd tslliaarng amdcvnslls ynanpsipnr qrrtaseyll eadkkphsll
421 qsnsnashsa fsfsgispai ispscsshaf vkaipsissk fsqlaeeyes qlrekeedli
481 ranrlkqdtl neisrtyqel tflqknnpty sqsmenlire aqetyqqlsk rlliwlearq
541 ifdlerslkp htslsisfps dflkkedgls lnndfkkpac nnvtnsdeye qlinkltslq
601 asrkkdtlyi rklyeelgid dtvnsyrrli amscginped lsleildave ealtrek
",
species_name = "Schizosaccharomyces pombe")
Now for YFO. Copy one of the samples above, edit it for the your Mbp1 homologue in YFO and add it to the database.
Then save the database, delete it and reload it:
save(db, file="proteinDB.RData") # write to file
rm(db) # remove
db # it's gone
load("proteinDB.RData") # read it back
db # verify
When that is done, we're ready to run some alignments.
Optimal Sequence Alignment at EMBOSS
Online programs for optimal sequence alignment are part of the EMBOSS tools. The programs take FASTA files or raw text files as input.
Local optimal sequence alignment using "water"
Task:
- Fetch the sequences for
MBP1_SACCE
andMBP1_YFORG
from your database. Something like:
getSeq(db, refseq_id = "NP_010227")
- Access the EMBOSS Explorer site (if you haven't done so yet, you might want to bookmark it.)
- Look for ALIGNMENT LOCAL, click on water, paste your sequences and run the program with default parameters.
- Study the results. You will probably find that the alignment extends over most of the protein, but does not include the termini.
- Considering the sequence identity cutoff we discussed in class (25% over the length of a domain), do you believe that the N-terminal domains (the APSES domains) are homologous?
- Change the Gap opening and Gap extension parameters to high values (e.g. 30 and 5). Then run the alignment again.
- Note what is different.
Global optimal sequence alignment using "needle"
Task:
- Look for ALIGNMENT GLOBAL, click on needle, paste the
MBP1_SACCE
andMBP1_YFORG
sequences again and run the program with default parameters. - Study the results. You will find that the alignment extends over the entire protein, likely with long indels at the termini.
The Mutation Data Matrix
The NCBI makes its alignment matrices available by ftp. They are located at ftp://ftp.ncbi.nih.gov/blast/matrices - for example here is a link to the BLOSUM62 matrix[2].
Scoring matrices are also available in the Bioconductor Biostrings package.
if (!require(Biostrings, quietly=TRUE)) {
source("https://bioconductor.org/biocLite.R")
biocLite("Biostrings")
library(Biostrings)
}
help(package = "Biostrings")
data(package = "Biostrings")
data(BLOSUM62)
BLOSUM62
A R N D C Q E G H I L K M F P S T W Y V B J Z X *
A 4 -1 -2 -2 0 -1 -1 0 -2 -1 -1 -1 -1 -2 -1 1 0 -3 -2 0 -2 -1 -1 -1 -4
R -1 5 0 -2 -3 1 0 -2 0 -3 -2 2 -1 -3 -2 -1 -1 -3 -2 -3 -1 -2 0 -1 -4
N -2 0 6 1 -3 0 0 0 1 -3 -3 0 -2 -3 -2 1 0 -4 -2 -3 4 -3 0 -1 -4
D -2 -2 1 6 -3 0 2 -1 -1 -3 -4 -1 -3 -3 -1 0 -1 -4 -3 -3 4 -3 1 -1 -4
C 0 -3 -3 -3 9 -3 -4 -3 -3 -1 -1 -3 -1 -2 -3 -1 -1 -2 -2 -1 -3 -1 -3 -1 -4
Q -1 1 0 0 -3 5 2 -2 0 -3 -2 1 0 -3 -1 0 -1 -2 -1 -2 0 -2 4 -1 -4
E -1 0 0 2 -4 2 5 -2 0 -3 -3 1 -2 -3 -1 0 -1 -3 -2 -2 1 -3 4 -1 -4
G 0 -2 0 -1 -3 -2 -2 6 -2 -4 -4 -2 -3 -3 -2 0 -2 -2 -3 -3 -1 -4 -2 -1 -4
H -2 0 1 -1 -3 0 0 -2 8 -3 -3 -1 -2 -1 -2 -1 -2 -2 2 -3 0 -3 0 -1 -4
I -1 -3 -3 -3 -1 -3 -3 -4 -3 4 2 -3 1 0 -3 -2 -1 -3 -1 3 -3 3 -3 -1 -4
L -1 -2 -3 -4 -1 -2 -3 -4 -3 2 4 -2 2 0 -3 -2 -1 -2 -1 1 -4 3 -3 -1 -4
K -1 2 0 -1 -3 1 1 -2 -1 -3 -2 5 -1 -3 -1 0 -1 -3 -2 -2 0 -3 1 -1 -4
M -1 -1 -2 -3 -1 0 -2 -3 -2 1 2 -1 5 0 -2 -1 -1 -1 -1 1 -3 2 -1 -1 -4
F -2 -3 -3 -3 -2 -3 -3 -3 -1 0 0 -3 0 6 -4 -2 -2 1 3 -1 -3 0 -3 -1 -4
P -1 -2 -2 -1 -3 -1 -1 -2 -2 -3 -3 -1 -2 -4 7 -1 -1 -4 -3 -2 -2 -3 -1 -1 -4
S 1 -1 1 0 -1 0 0 0 -1 -2 -2 0 -1 -2 -1 4 1 -3 -2 -2 0 -2 0 -1 -4
T 0 -1 0 -1 -1 -1 -1 -2 -2 -1 -1 -1 -1 -2 -1 1 5 -2 -2 0 -1 -1 -1 -1 -4
W -3 -3 -4 -4 -2 -2 -3 -2 -2 -3 -2 -3 -1 1 -4 -3 -2 11 2 -3 -4 -2 -2 -1 -4
Y -2 -2 -2 -3 -2 -1 -2 -3 2 -1 -1 -2 -1 3 -3 -2 -2 2 7 -1 -3 -1 -2 -1 -4
V 0 -3 -3 -3 -1 -2 -2 -3 -3 3 1 -2 1 -1 -2 -2 0 -3 -1 4 -3 2 -2 -1 -4
B -2 -1 4 4 -3 0 1 -1 0 -3 -4 0 -3 -3 -2 0 -1 -4 -3 -3 4 -3 0 -1 -4
J -1 -2 -3 -3 -1 -2 -3 -4 -3 3 3 -3 2 0 -3 -2 -1 -2 -1 2 -3 3 -3 -1 -4
Z -1 0 0 1 -3 4 4 -2 0 -3 -3 1 -1 -3 -1 0 -1 -2 -2 -2 0 -3 4 -1 -4
X -1 -1 -1 -1 -1 -1 -1 -1 -1 -1 -1 -1 -1 -1 -1 -1 -1 -1 -1 -1 -1 -1 -1 -1 -4
* -4 -4 -4 -4 -4 -4 -4 -4 -4 -4 -4 -4 -4 -4 -4 -4 -4 -4 -4 -4 -4 -4 -4 -4 1
BLOSUM62["H", "H"]
BLOSUM62["L", "L"]
BLOSUM62["S", "T"]
BLOSUM62["L", "D"]
Task:
- Study this and make sure you understand what this table is, how it can be used, and what a reasonable range of values for identities and pairscores for non-identical, similar and dissimilar residues is. Ask on the mailing list in case you have questions.
Alignment with Biostrings
Biostrings has extensive functions for sequence alignments. They are generally well written and tightly integrated with the rest of Bioconductor's functions. There are a few quirks however: for example alignments won't work with lower-case sequences. This is why our getSeq()
changes sequences to uppercase.
# sequence are stored in AAstring objects
?AAString
seq1 <- AAString(getSeq(db, refseq_id = "NP_010227"))
seq2 <- AAString(getSeq(db, refseq_id = "NP_593032")) # use MBP1_YFORG instead!
?pairwiseAlignment
# global alignment with end-gap penalties is default.
ali1 <- pairwiseAlignment(
seq1,
seq2,
substitutionMatrix = "BLOSUM62",
gapOpening = 10,
gapExtension = 0.5)
writePairwiseAlignments(ali1)
# local alignment
ali2 <- pairwiseAlignment(
seq1,
seq2,
type = "local",
substitutionMatrix = "BLOSUM62",
gapOpening = 50,
gapExtension = 10)
writePairwiseAlignments(ali2)
Task:
Have a look at the two alignments. Compare. The local alignment is weighted heavily to an indel-free alignment by setting very high gap penalties. Try changing them and see what happens.
BLAST
BLAST is by a margin the most important computational tool of molecular biology. It is so important, that we have already used BLAST in Assignment 2 even before properly introducing the algorithm and the principles, to find the most similar sequence to MBP1_SACCE
in YFO.
In this part of the assignment we will use BLAST to perform Reciprocal Best Matches.
One of the important questions of model-organism based inference is: which genes perform the same function in two different organisms. In the absence of other information, our best guess is that these are the two genes that are mutually most similar. The keyword here is mutually. If MBP1_SACCE
from S. cerevisiae is the best match to RES2_SCHPO
in S. pombe, the two proteins are only mutually most similar if RES2_SCHPO
is more similar to MBP1_SACCE
than to any other S. cerevisiae protein. We call this a Reciprocal Best Match, or "RBM"[3].
The argument is summarized in the figure on the right: genes that evolve under continuos selective pressure on their function have relatively lower mutation rates and are thus more similar to each other, than genes that undergo neo- or sub- functionalization after duplication.
Proteins are often composed of multiple domains that represent distinct roles in a gene's function. Under the assumptions above we could hypothesize:
- a gene in YFO that has the "same" function as the Mbp1 cell-cycle checkpoint switch in yeast should be an RBM to Mbp1;
- a gene that binds to the same DNA sites as Mbp1 should have a DNA-binding domain that is an RBM to the DNA binding domain of Mbp1.
Thus we'll compare RBMs in YFO for full-length Mbp1_SACCE
and its DNA-binding domain, and see if the results are the same.
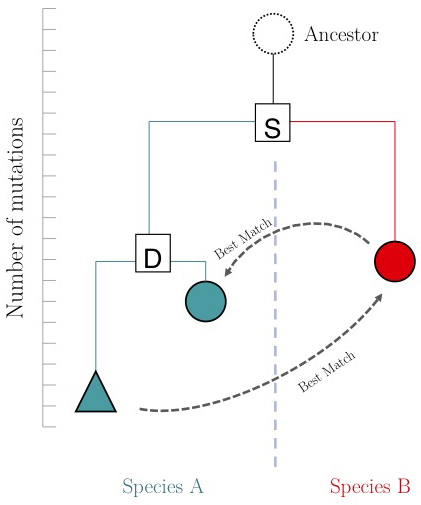
Full-length RBM
You have already performed the first half of the experiment: matching from S. cerevisiae to YFO. The backward match is simple.
Task:
- Access BLAST and follow the link to the protein blast program.
- Enter the refseq ID for
MBP1_YFORG
in the Query sequence field. - Select
refseq_protein
as the database to search in, and enterSaccharomyces cerevisiae (taxid:4932)
to restrict the organism for which hits are reported. - Run BLAST. Examine the results.
If your top-hit is NP_010227
, you have confirmed the RBM between Mbp1_SACCE
and Mbp1_YFORG
. If it is not, let me know. I expect this to be the same and would like to verify your results if it is not.
RBM for the DNA binding domain
The DNA-binding domain of Mbp1_SACCE
is called an APSES domain.
Defining the domain sequence
The APSES domain is a well-defined type of DNA-binding domain that is ubiquitous in fungi and unique in that kingdom. Structurally it is a member of the Winged Helix-Turn-Helix family. Recently it was found that it is homologous to the somewhat shorter, prokaryotic KilA-N domain; thus the APSES domain was retired from pFam and instances were merged into the KilA-N family. However InterPro has a KilA-N entry but still recognizes the APSES domain.
KilA-N domain boundaries in Mbp1 can be derived from the results of a CDD search with the ID 1BM8_A (the Mbp1 DNA binding domain crystal structure). The KilA-N superfamily domain alignment is returned.
- (pfam 04383): KilA-N domain; The amino-terminal module of the D6R/N1R proteins defines a novel, conserved DNA-binding domain (the KilA-N domain) that is found in a wide range of proteins of large bacterial and eukaryotic DNA viruses. The KilA-N domain family also includes the previously defined APSES domain. The KilA-N and APSES domains may also share a common fold with the nucleic acid-binding modules of the LAGLIDADG nucleases and the amino-terminal domains of the tRNA endonuclease.
10 20 30 40 50 60 70 80
....*....|....*....|....*....|....*....|....*....|....*....|....*....|....*....|
1BM8A 16 IHSTGSIMKRKKDDWVNATHILKAANFAKaKRTRILEKEVLKETHEKVQ---------------GGFGKYQGTWVPLNIA 80
Cdd:pfam04383 3 YNDFEIIIRRDKDGYINATKLCKAAGETK-RFRNWLRLESTKELIEELSeennvdkseiiigrkGKNGRLQGTYVHPDLA 81
90
....*....|....
1BM8A 81 KQLA----EKFSVY 90
Cdd:pfam04383 82 LAIAswisPEFALK 95
Note that CDD and SMART are not consistent in how they apply pFam 04383
to the Mbp1 sequence. See annotation below.
The CDD KilA-N domain definition begins at position 16 of the 1BM8 sequence. But virtually all fungal APSES domains have a longer, structurally defined, conserved N-terminus. Blindly applying the KilA-N domain definition to these proteins would lose important information. For most purposes we will prefer the sequence spanned by the 1BM8_A structure. The sequence is given below, the KilA-N domain is coloured dark green. By this definition the APSES domain is 99 amino acids long and comprises residues 4 to 102 of the NP_010227
sequence.
10 20 30 40 50 60 70 80
....*....|....*....|....*....|....*....|....*....|....*....|....*....|....*....|
1BM8A 1 QIYSARYSGVDVYEFIHSTGSIMKRKKDDWVNATHILKAANFAKAKRTRILEKEVLKETHEKVQGGFGKYQGTWVPLNIA 80
90
....*....|....*....
1BM8A 81 KQLAEKFSVYDQLKPLFDF 99
- Yeast APSES domain sequence in FASTA format
>APSES_MBP1 Residues 4-102 of S. cerevisiae Mbp1 QIYSARYSGVDVYEFIHSTGSIMKRKKDDWVNATHILKAANFAKAKRTRI LEKEVLKETHEKVQGGFGKYQGTWVPLNIAKQLAEKFSVYDQLKPLFDF
- Synopsis of ranges
Domain | Link | Length | Boundary | Range (Mbp1) | Range (1BM8) |
KilA-N: pfam04383 (CDD) | CDD alignment | 72 | STGSI ... KFSVY | 21 - 93 | 18 - 90 |
KilA-N: pfam04383 (SMART) | Smart main page | 79 | IHSTG ... YDQLK | 19 - 97 | 16 - 94 |
KilA-N: SM01252 (SMART) | Smart main page | 84 | TGSIM ... DFTQT | 22 - 105 | 19 - 99... |
APSES: Interpro IPR003163 | (Interpro) | 130 | QIYSA ... IRSAS | 3 - 133 | 1 - 99... |
APSES (1BM8) | – | 99 | QIYSA ... PLFDF | 4 - 102 | 1 - 99 |
Executing the forward search
Task:
- Access BLAST and follow the link to the protein blast program.
- Forward search:
- Enter only the APSES domain sequence for
MBP1_YFORG
in the Query sequence field (copied from above). - Select
refseq_protein
as the database to search in, and enter the correct taxonomy ID for YFO. - Run BLAST. Examine the results.
- If this is the same protein you have already seen, oK. If it's not add it to your protein database.
- Enter only the APSES domain sequence for
Alignment to define the sequence for the reverse search
Task:
- Define the YFO best-match APSES sequence by performing a global, optimal sequence alignment of the yeast domain with the full length protein sequence of your BLAST hit. Align these two sequences of very different length without end-gap penalties. Here is sample code that you can adapt.
# Align the yeast Mbp1 APSES domain with another protein sequence.
# Pattern:
apses <- AAString(in2seq("QIYSARYSGVDVYEFIHSTGSIMKRKKDDWVNATHILKAANFAKAKRTRI
LEKEVLKETHEKVQGGFGKYQGTWVPLNIAKQLAEKFSVYDQLKPLFDF"))
# Query:
# (Obviously, use the YFO best hit sequence instead of SCHPO...)
blastHit <- AAString(getSeq(db, refseq_id = "NP_593032"))
# This alignment uses the "overlap" type. "overlap" turns the
# end-gap penalties off and that is crucially important since
# the sequences have very different length.
aliApses <- pairwiseAlignment(
apses,
blastHit,
type = "overlap",
substitutionMatrix = "BLOSUM62",
gapOpening = 10,
gapExtension = 0.5)
# Inspect the result. The aligned sequences should be clearly
# homologous, and have (almost) no indels. The entire "pattern"
# sequence from QIYSAR ... to ... KPLFDF should be matched
# with the "query".
writePairwiseAlignments(aliApses)
# If this is correct, you can extract the matched sequence from
# the alignment object. The syntax is a bit different from what
# you have seen before: this is an "S4 object", not a list. No
# worries: as.character() returns a normal string.
as.character(aliApses@subject)
Executing the reverse search
Task:
- Copy the the APSES domain sequence for the YFO best-match and enter it into Query sequence field of the BLAST form.
- Select
refseq_protein
as the database to search in, and enterSaccharomyces cerevisiae (taxid:4932)
to restrict the organism for which hits are reported. - Run BLAST. Examine the results.
- Select
If your top-hit is again NP_010227
, you have confirmed the RBM between the APSES domain of Mbp1_SACCE
and Mbp1_YFORG
. If it is not, let me know. There may be some organisms for which the full-length and APSES RBMs are different and I would like to discuss these cases.
PSI BLAST
It is (deceptively) easy to perform BLAST searches via the Web interface, but to use such powerful computational tools to their greatest advantage takes a considerable amount of care, caution and consideration.
PSI-BLAST allows to perform very sensitive searches for homologues that have diverged so far that their pairwise sequence similarity has become insignificant. It achieves this by establishing a profile of sequences to align with the database, rather than searching with individual sequences. This deemphasizes parts of the sequence that are variable and inconsequential, and focusses on the parts of greater structural and functional importance. As a consequence, the signal to noise ratio is greatly enhanced.
In this part of the assignment, we will set ourselves the task to use PSI-BLAST and find all orthologs and paralogs of the APSES domain containing transcription factors in YFO. We will use these sequences for multiple alignments, calculation of conservation etc.
The first methodical problem we have to address is what sequence to search with. The full-length Mbp1 sequence from Saccharomyces cerevisiae or its RBM from YFO are not suitable: They contain multiple domains (in particular the ubiquitous Ankyrin domains) and would create broad, non-specific profiles. The APSES domain sequence by contrast is structurally well defined. The KilA-N domain, being shorter, is less likely to make a sensitive profile. Indeed one of the results of our analysis will be to find whether APSES domains in fungi all have the same length as the Mbp1 domain, or whether some are indeed much shorter, like the KILA-N domain, as suggested by the Pfam alignment.
The second methodical problem we must address is how to perform a sensitive PSI-BLAST search in one organism. We need to balance two conflicting objectives:
- If we restrict the PSI-BLAST search to YFO, PSI-BLAST has little chance of building a meaningful profile - the number of homologues that actually are in YFO is too small. Thus the search will not become very sensitive.
- If we don't restrict our search, but search in all species, the number of hits may become unwieldily large. It becomes increasingly difficult to closely check all hits as to whether they have good coverage. Also we need to evaluate the fringe cases of marginal E-value: should a new sequence be added to the profile, or should we hold off on it for one or two iterations, to see whether its E-value drops significantly. By all means, we need to avoid profile corruption.
Perhaps this is still be manageable when we are searching in fungi, but imagine you are working with a bacterial protein, or a protein that is conserved across the entire tree of life: your search may find tens of thousands of sequences. And by next year, thousands more will have been added.
Therefore we have to find a middle ground: add enough organisms (sequences) to compile a sensitive profile, but not so many that we can no longer individually assess the sequences that contribute to the profile. We need to define a broadly representative but manageable set of species - to exploit the transitivity of homology - even if we are interested only in matches in one species: YFO. Please reflect on this and make sure you understand why we include sequences in a PSI-BLAST search that we are not actually interested in.
We need a subset of species
- that represent as large a range as possible on the evolutionary tree;
- that are as well distributed as possible on the tree; and
- whose genomes are fully sequenced.
Selecting species for a PSI-BLAST search
To selct species, we will use an approach that is conceptually simple: select a set of species according to their shared taxonomic rank in the tree of life. Biological classification provides a hierarchical system that describes evolutionary relatedness for all living entities. The levels of this hierarchy are so called taxonomic ranks. These ranks are defined in Codes of Nomenclature that are curated by the self-governed international associations of scientists working in the field. The number of ranks is not specified: there is a general consensus on seven principal ranks (see below, in bold) but many subcategories exist and may be newly introduced. It is desired–but not mandated–that ranks represent clades (a group of related species, or a "branch" of a phylogeny), and it is desired–but not madated–that the rank is sharply defined. The system is based on subjective dissimilarity. Needless to say that it is in flux.
If we follow a link to an entry in the NCBI's Taxonomy database, eg. Saccharomyces cerevisiae S228c, the strain from which the original "yeast genome" was sequenced in the late 1990s, we see the following specification of its taxonomic lineage:
cellular organisms; Eukaryota; Opisthokonta;
Fungi; Dikarya; Ascomycota; Saccharomyceta;
Saccharomycotina; Saccharomycetes;
Saccharomycetales; Saccharomycetaceae;
Saccharomyces; Saccharomyces cerevisiae
These names can be mapped into taxonomic ranks, since the suffixes of these names e.g. -mycotina, -mycetaceae are specific to defined ranks. (NCBI does not provide this mapping, but Wikipedia is helpful here.)
Rank | Suffix | Example |
Domain | Eukaryota (Eukarya) | |
Subdomain | Opisthokonta | |
Kingdom | Fungi | |
Subkingdom | Dikarya | |
Phylum | Ascomycota | |
rankless taxon[4] | -myceta | Saccharomyceta |
Subphylum | -mycotina | Saccharomycotina |
Class | -mycetes | Saccharomycetes |
Subclass | -mycetidae | |
Order | -ales | Saccharomycetales |
Family | -aceae | Saccharomycetaceae |
Subfamily | -oideae | |
Tribe | -eae | |
Subtribe | -ineae | |
Genus | Saccharomyces | |
Species | Saccharomyces cerevisiae |
You can see that there is no common mapping between the yeast lineage listed at the NCBI and the commonly recognized categories - not all ranks are represented. Nor is this consistent across species in the taxonomic database: some have subfamily ranks and some don't. And the tree is in no way normalized - some of the ranks have thousands of members, and for some, only a single extant member may be known, or it may be a rank that only relates to the fossil record.
But the ranks do provide some guidance to evolutionary divergence. Say you want to choose four species across the tree of life for a study, you should choose one from each of the major domains of life: Eubacteria, Euryarchaeota, Crenarchaeota-Eocytes, and Eukaryotes. Or you want to study a gene that is specific to mammals. Then you could choose from the clades listed in the NCBI taxonomy database under Mammalia (a class rank, and depending how many species you would want to include, use the subclass-, order-, or family rank (hover over the names to see their taxonomic rank.)
After a fair bit of manual experimentation I have picked the 16 species below to define a well-distributed search-space for PSI-BLAST. Of course you must also include YFO in the selection (if YFO is not in this list already).
To enter these 16 species as an Entrez restriction, they need to be formatted as below. (One could also enter species one by one, by pressing the (+) button after the organism list)
"Aspergillus nidulans"[orgn]
OR "Glarea lozoyensis ATCC 20868"[orgn]
OR "Cryptococcus neoformans var. neoformans JEC21]"[orgn]
OR "Colletotrichum graminicola"[orgn]
OR "Magnaporthe oryzae 70-15"[orgn]
OR "Melampsora larici-populina 98AG31"[orgn]
OR "Neurospora crassa"[orgn]
OR "Phaeosphaeria nodorum"[orgn]
OR "Puccinia graminis"[orgn]
OR "Sclerotinia sclerotiorum"[orgn]
OR "Trichoderma reesei"[orgn]
OR "Tuber melanosporum"[orgn]
OR "Saccharomyces cerevisiae S288c"[orgn]
OR "Schizosaccharomyces pombe 972h-"[orgn]
OR "Ustilago maydis 521"[orgn]
OR "Zymoseptoria tritici"[orgn]
Executing the PSI-BLAST search
We have a list of species. Good. Next up: how do we use it.
Task:
- Navigate to the BLAST homepage.
- Select protein BLAST.
- Paste the APSES domain sequence into the search field.
- Select refseq as the database.
- Copy the Entrez restrictions from above and enter the correct name for YFO into the list if it is not there already. (Obviously, you can't find sequences in YFO if YFO is not included among your organisms.) Paste the list into the Entrez Query field.
- In the Algorithm section, select PSI-BLAST.
- Click on BLAST.
Evaluate the results carefully. Since we did not change the algorithm parameters, the threshold for inclusion was set at an E-value of 0.005 by default, and that may be a bit too lenient, i.e. include sequences that are not homologous. If you look at the table of your hits– in the Sequences producing significant alignments... section– there may also be a few sequences that have a low query coverage of less than 80%. Let's exclude these from the profile initially: not to worry, if they are true positives, the will come back with improved E-values and greater coverage in subsequent iterations. But if they were false positives, their E-values will rise and they should drop out of the profile and not contaminate it.
Task:
- In the header section, click on Formatting options and in the line "Format for..." set the with inclusion threshold to
0.001
(This means E-values can't be above 10-03 for the sequence to be included.) - Click on the Reformat button (top right).
- In the table of sequence descriptions (not alignments!), click on Query cover to sort the table by coverage, not by score.
- Deselect the check mark next to these sequences in the second-to-rightmost column Select for PSI blast.
- Then scroll to Run PSI-BLAST iteration 2 ... and click on
Go
.
This is now the "real" PSI-BLAST at work: it constructs a profile from all the full-length sequences and searches with the profile, not with any individual sequence. Note that we are controlling what goes into the profile in two ways:
- we are explicitly removing sequences with poor coverage; and
- we are requiring a more stringent minimum E-value for each sequence.
Task:
- Again, study the table of hits. Sequences highlighted in yellow have met the search criteria in the second iteration and are proposed for inclusion in the next iteration. Note that the coverage of (some) of the previously excluded sequences is now above 80%. These are the ones you need to check carefully: do you agree that they should be included? If there is any doubt, perhaps because of a really marginal E-value, poor coverage or a function annotation that is not compatible with your query, it is safer to exclude a sequence than to risk profile corruption. If the sequence is a true positive, it will return to the list in later iterations, usually with a better E-value as the profile improves. It's a good idea to note such sequences in your journal so you can keep track of how their E-values change.
- Let's exclude partial matches one more time. Again, deselect all sequences with less than 80% coverage. Then run the third iteration.
- Iterate the search in this way until no more "New" sequences are added to the profile. The search has converged.
- Now look at the list of excluded hits (if any), the hits that are reasonable but didn't quite make the cut. Are there any from YFO that seem like they should actually be included? Perhaps their E-value is only marginally above the threshold? If that's the case, try returning the E-value threshold to the default 0.005 and see what happens...
Once no "new" sequences have been added, we would always get the same result on additional iterations because there are no more changes to the profile. We say that the search has converged. Time to harvest.
Task:
- At the header, click on Taxonomy reports and find YFO in the Organism Report section. These are your APSES domain homologs. All of them. Actually, perhaps more than all: the report may also include sequences with E-values above the inclusion threshold.
- From the report copy the sequence identifiers from YFO, with E-values above your defined threshold to your notebook.
For example, the list of Saccharomyces genes is the following:
Saccharomyces cerevisiae S288c [ascomycetes] taxid 559292
ref|NP_010227.1| Mbp1p [Saccharomyces cerevisiae S288c] [ 131] e-36
ref|NP_011036.1| Swi4p [Saccharomyces cerevisiae S288c] [ 123] 1e-27
ref|NP_012881.1| Phd1p [Saccharomyces cerevisiae S288c] [ 91] 1e-24
ref|NP_013729.1| Sok2p [Saccharomyces cerevisiae S288c] [ 93] 2e-24
ref|NP_012165.1| Xbp1p [Saccharomyces cerevisiae S288c] [ 40] 5e-05
Xbp1 is a special case. It has only very low coverage, but that is because it has a long domain insertion and the N-terminal match often is not recognized by alignment because the gap scores for long indels are unrealistically large. For now, I keep that sequence with the others.
Task:
- To add the sequences to your database, open each of the links for an organism into a separate tab.
- Create an R-script and compile an
addToDB
statement for each of your proteins. I find all this information on the Genbank record itself, and on the "Identical Proteins" page linked to it. - Execute the script.
db <- addToDB(db,
name = "Mbp1",
refseq_id = "NP_010227",
uniprot_id = "P39678",
taxonomy_id = 4932,
genome_xref = "NC_001136.10",
genome_from = 352877,
genome_to = 355378,
sequence = "
1 msnqiysary sgvdvyefih stgsimkrkk ddwvnathil kaanfakakr trilekevlk
61 ethekvqggf gkyqgtwvpl niakqlaekf svydqlkplf dftqtdgsas pppapkhhha
121 skvdrkkair sastsaimet krnnkkaeen qfqsskilgn ptaaprkrgr pvgstrgsrr
181 klgvnlqrsq sdmgfprpai pnssisttql psirstmgpq sptlgileee rhdsrqqqpq
241 qnnsaqfkei dledglssdv epsqqlqqvf nqntgfvpqq qssliqtqqt esmatsvsss
301 pslptspgdf adsnpfeerf pgggtspiis miprypvtsr pqtsdindkv nkylsklvdy
361 fisnemksnk slpqvllhpp phsapyidap idpelhtafh wacsmgnlpi aealyeagts
421 irstnsqgqt plmrsslfhn sytrrtfpri fqllhetvfd idsqsqtvih hivkrksttp
481 savyyldvvl skikdfspqy rielllntqd kngdtalhia skngdvvffn tlvkmgaltt
541 isnkegltan eimnqqyeqm miqngtnqhv nssntdlnih vntnnietkn dvnsmvimsp
601 vspsdyityp sqiatnisrn ipnvvnsmkq masiyndlhe qhdneikslq ktlksisktk
661 iqvslktlev lkesskdeng eaqtnddfei lsrlqeqntk klrkrliryk rlikqkleyr
721 qtvllnklie detqattnnt vekdnntler lelaqeltml qlqrknklss lvkkfednak
781 ihkyrriire gtemnieevd ssldvilqtl iannnknkga eqiitisnan sha
",
species_name = "Saccharomyces cerevisiae")
db <- addToDB(db,
name = "Swi4",
refseq_id = "NP_011036",
uniprot_id = "P25302",
taxonomy_id = 4932,
genome_xref = "NC_001137.3",
genome_from = 385876,
genome_to = 382595,
sequence = "
1 mpfdvlisnq kdntnhqnit pisksvllap hsnhpvieia tysetdvyec yirgfetkiv
61 mrrtkddwin itqvfkiaqf sktkrtkile kesndmqhek vqggygrfqg twipldsakf
121 lvnkyeiidp vvnsiltfqf dpnnpppkrs knsilrktsp gtkitspssy nktprkknss
181 sstsatttaa nkkgkknasi nqpnpsplqn lvfqtpqqfq vnssmnimnn ndnhttmnfn
241 ndtrhnlinn isnnsnqsti iqqqksihen sfnnnysatq kplqffpipt nlqnknvaln
301 npnnndsnsy shnidnvins snnnnngnnn nliivpdgpm qsqqqqqhhh eyltnnfnhs
361 mmdsitngns kkrrkklnqs neqqfynqqe kiqrhfklmk qpllwqsfqn pndhhneycd
421 sngsnnnnnt vasngssiev fssnendnsm nmssrsmtpf sagntssqnk lenkmtdqey
481 kqtiltilss erssdvdqal latlypapkn fninfeiddq ghtplhwata maniplikml
541 itlnanalqc nklgfncitk sifynncyke nafdeiisil kiclitpdvn grlpfhylie
601 lsvnksknpm iiksymdsii lslgqqdynl lkiclnyqdn igntplhlsa lnlnfevynr
661 lvylgastdi lnldnespas imnkfntpag gsnsrnnntk adrklarnlp qknyyqqqqq
721 qqqpqnnvki pkiiktqhpd kedstadvni aktdsevnes qylhsnqpns tnmntimedl
781 sninsfvtss vikdikstps kilenspily rrrsqsisde kekakdnenq vekkkdplns
841 vktampsles pssllpiqms plgkyskpls qqinklntkv sslqrimgee iknldnevve
901 tessisnnkk rlitiahqie dafdsvsnkt pinsisdlqs riketsskln sekqnfiqsl
961 eksqalklat ivqdeeskvd mntnssshpe kqedeepipk stsetsspkn tkadakfsnt
1021 vqesydvnet lrlateltil qfkrrmttlk iseakskins svkldkyrnl igitienids
1081 klddiekdlr ana
",
species_name = "Saccharomyces cerevisiae")
db <- addToDB(db,
name = "Phd1",
refseq_id = "NP_012881",
uniprot_id = "P36093",
taxonomy_id = 4932,
genome_xref = "NC_001143.9",
genome_from = 356748,
genome_to = 357848,
sequence = "
1 myhvpemrlh yplvntqsna aitptrsydn tlpsfnelsh qstinlpfvq retpnayanv
61 aqlatsptqa ksgyycryya vpfptypqqp qspyqqavlp yatipnsnfq pssfpvmavm
121 ppevqfdgsf lntlhphtel ppiiqntndt svarpnnlks iaaasptvta ttrtpgvsst
181 svlkprvitt mwedenticy qveangisvv rradnnming tkllnvtkmt rgrrdgilrs
241 ekvrevvkig smhlkgvwip ferayilaqr eqildhlypl fvkdiesivd arkpsnkasl
301 tpksspapik qepsdnkhei ateikpksid alsngastqg agelphlkin hidteaqtsr
361 aknels
",
species_name = "Saccharomyces cerevisiae")
db <- addToDB(db,
name = "Sok2",
refseq_id = "NP_013729",
uniprot_id = "P53438",
taxonomy_id = 4932,
genome_xref = "NC_001145.3",
genome_from = 305593,
genome_to = 303236,
sequence = "
1 mpignpintn diksnrmrqe snmsavsnse stigqstqqq qqqqqylgqs vqplmpvsyq
61 yvvpeqwpyp qyyqqpqsqs qqqlqsqpqm yqvqesfqss gsdsnasnpp stsvgvpsna
121 tatalpngsa ittkksnnst nisnnvpyyy yfpqmqaqqs maysypqayy yypangdgtt
181 ngatpsvtsn qvqnpnlekt ystfeqqqqh qqqqqlqaqt ypaqppkign afskfsksgp
241 psdsssgsms pnsnrtsrns nsisslaqqp pmsnypqpst yqypgfhkts sipnshspip
301 prslttptqg ptsqngplsy nlpqvgllpp qqqqqvsply dgnsitppvk pstdqetylt
361 anrhgvsdqq ydsmaktmns fqtttirhpm pliattnatg sntsgtsasi irprvtttmw
421 edektlcyqv eangisvvrr adndmvngtk llnvtkmtrg rrdgilkaek irhvvkigsm
481 hlkgvwipfe ralaiaqrek iadylyplfi rdiqsvlkqn npsndsssss sstgiksisp
541 rtyyqpinny qnpngpsnis aaqltyssmn lnnkiipnns ipavstiaag ekplkkctmp
601 nsnqleghti tnlqtlsatm pmkqqlmgni asplsyprna tmnsastlgi tpadskpltp
661 sptttntnqs sesnvgsiht gitlprvese sashskwske adsgntvpdn qtlkeprssq
721 lpisaltstd tdkiktstsd eatqpnepse aepvkesess ksqvdgagdv sneeiaaddt
781 kkqek
",
species_name = "Saccharomyces cerevisiae")
db <- addToDB(db,
name = "Xbp1",
refseq_id = "NP_012165",
uniprot_id = "P40489",
taxonomy_id = 4932,
genome_xref = "NC_001141.2",
genome_from = 177250,
genome_to = 175307,
sequence = "
1 mkypafsins dtvhltdnpl ddyqrlylvs vldrdsppas fsaglnirkv nykssiaaqf
61 thpnfiisar dagngeeaaa qnvlncfeyq fpnlqtiqsl vheqtllsql assatphsal
121 hlhdknilmg kiilpsrsnk tpvsasptkq ekkalstasr enatssltkn qqfkltkmdh
181 nlindklinp nncviwshds gyvfmtgiwr lyqdvmkgli nlprgdsvst sqqqffckae
241 fekilsfcfy nhssftsees ssvllsssts sppkrrtstg stfldanass sstsstqann
301 yidfhwnnik pelrdlicqs ykdflinelg pdqidlpnln panftkrirg gyikiqgtwl
361 pmeisrllcl rfcfpiryfl vpifgpdfpk dceswylahq nvtfassttg agaataataa
421 antstnftst avarprqkpr prprqrstsm shskaqklvi edalpsfdsf venlglssnd
481 knfikknskr qksstytsqt sspigprdpt vqilsnlasf ynthghrysy pgniyipqqr
541 yslpppnqls spqrqlnyty dhihpvpsqy qsprhynvps spiapapptf pqpygddhyh
601 flkyasevyk qqnqrpahnt ntnmdtsfsp rannslnnfk fktnskq
",
species_name = "Saccharomyces cerevisiae")
So much for using PSI-BLAST. The last step seems a bit tedious, adding all this information by hand. There's got to be a better way, right?
But for now, we'll have a look at what the sequences tell us.
- TBC
Links and resources
Footnotes and references
- ↑ This is not strictly true in all cases: some algorithms measure similarity through an alignment-free approach, for example by comparing structural features, or domain annotations. However, these methods are mostly only important when sequences are so highly diverged that no meaningful alignment can be produced.
- ↑ That directory also contains sourcecode to generate the PAM matrices. This may be of interest if you ever want to produce scoring matrices from your own datasets.
- ↑ Note that RBMs are usually orthologues, but the definition of orthologue and RBM is not the same. Most importantly, many orthologues are not RBMs. We will explore this more when we discuss phylogenetic inference.
- ↑ The -myceta are well supported groups above the Class rank. See Leotiomyceta for details and references.
Ask, if things don't work for you!
- If anything about the assignment is not clear to you, please ask on the mailing list. You can be certain that others will have had similar problems. Success comes from joining the conversation.
- Do consider how to ask your questions so that a meaningful answer is possible:
- How to create a Minimal, Complete, and Verifiable example on stackoverflow and ...
- How to make a great R reproducible example are required reading.
< Assignment 3 | Assignment 5 > |