Difference between revisions of "BIO Assignment Week 4"
m |
|||
Line 734: | Line 734: | ||
# Inspect the result. The aligned sequences should be clearly | # Inspect the result. The aligned sequences should be clearly | ||
− | # homologous, and have (almost) no indels. The entire | + | # homologous, and have (almost) no indels. The entire "pattern" |
− | # from QIYSAR ... to ... KPLFDF should be matched. | + | # sequence from QIYSAR ... to ... KPLFDF should be matched |
+ | # with the "query". | ||
writePairwiseAlignments(aliApses) | writePairwiseAlignments(aliApses) | ||
# If this is correct, you can extract the matched sequence from | # If this is correct, you can extract the matched sequence from | ||
− | # the alignment object. The syntax is a bit different | + | # the alignment object. The syntax is a bit different from what |
# you have seen before: this is an "S4 object", not a list. No | # you have seen before: this is an "S4 object", not a list. No | ||
# worries: as.character() returns a normal string. | # worries: as.character() returns a normal string. |
Revision as of 22:07, 11 October 2015
Assignment for Week 4
Sequence alignment
< Assignment 3 | Assignment 5 > |
Note! This assignment is currently active. All significant changes will be announced on the mailing list.
Concepts and activities (and reading, if applicable) for this assignment will be topics on next week's quiz.
Contents
- Take care of things, and they will take care of you.
- Shunryu Suzuki
Introduction
Sequence alignment is a very large, and important topic. One of the foundations of bioinformatics is the empirical observation that related sequences conserve structure, and often function. This is the basis on which we can make inferences from well-studied model organisms in species that have not been studied as deeply. And the foundation of discovering relatedness is to measure protein sequence similarity. If two sequences are much more similar than we could expect from chance, we hypothesize that their similarity comes from shared ancestry. The measurement of sequence similarity however requires sequence alignment[1].
In this assignment we will explore the essentials of
- optimal global and local alignment;
- BLAST searches for best matches;
- PSI BLAST searches for exhaustive matches; and
- Multiple sequence alignments.
As usual, the focus will be on practical, hands on approaches.
This is the scenario in brief: you have identified a best match for a Mbp1 relative in YFO. How can you identify all related genes in YFO? And, what can you learn from this collection?
Optimal sequence alignments
Let's start by aligning the sequences of Mbp1 and the YFO relative. For simplicity, I will call the two proteins MBP1_SACCE
and MBP1_YFORG
through the remainder of the assignment, and even if I casually refer to a gene when I'm really talking about a protein (sorry), you should recognize from context what is meant.
Preparation: Updated Database Functions
First we need to pull out the two sequences from the database object we created last time. You could recreate the state of your database by re-running the relevant parts of the script, or piece things together from the code of the previous assignment.
Keeping things in scripts is really useful.
But since we'll be working more with our database, adding to the data model, updating code for getting and setting data, and adding proteins, annotations and cross-references, let's spend a moment to organize things in a more principled way.
- We should create a script that loads the functions to manage the database;
- We should save our database so we can easily reload the contents.
Task:
Here's how we should organize this:
- We'll define a variable called
PROJECTDIR
which automatically gets set whenever you startup R. - A scriptfile with the necessary functions should automatically get
source()
'd at startup; - The database should be saved so it can easily be loaded.
You will find the code below. It looks long, but it's really quite straightforward bookkeeping. I have added a number of tests to help make sure the input is sane. That actually makes up the majority of the code. Sanitizing user input is always much more effort than the actual algorithm. I have tested the functions and think they should work as expected. But if you come across a situation where your input produces an error, or creates an inconsistency in the database, by all means let me know so the code can be improved.
1. Create a project directory for the assignments on your computer if you don't have one yet.
2. Adapt the code below as needed, and execute it to update .Rprofile
.
file.edit("~/.Rprofile")
# Add:
PROJECTDIR <- "full/path/to/your/directory/" # including the final backslash.
source(paste(PROJECTDIR, "dbUtilities.R", sep=""))
# ... and save the file.
# To make the definition available, run it.
source("~/.Rprofile")
# Now let's create the script for the database functions:
file.edit(paste(PROJECTDIR, "dbUtilities.R", sep=""))
An edit window for the file has opened. Copy the entire code block below, and paste it into the editor.
# dbUtilities.R
#
# Purpose: Utility functions for a Protein datamodel
# Version: 0.1
# Date: Oct 2015
# Author: Boris and class
#
# ToDo: Add more tables.
# Accept either taxonomy_id OR species name
# and pull the other from NCBI.
# Notes: Cf. schema sketch at
# http://steipe.biochemistry.utoronto.ca/abc/index.php/File:ProteinDataModel.1.jpg
# Currently implements only "protein" and
# "taxonomy" table.
# ==========================================================
# ==== FUNCTIONS =========================================
# ==== createDB =============================================
# Returns an empty list
# We use a separate function because we might want to
# some initialization code later.
createDB <- function() {
return(list())
}
# ==== in2seq ==============================================
# Utility function to sanitize input and convert it into a
# sequence string. Case can be optionally changed.
# Letters that are not one-letter code - such as
# ambiguity codes - throw an error if not explicitly
# permitted.
in2seq <- function(s, uc = FALSE, lc = FALSE, noAmbig = TRUE) {
s <- paste(unlist(s), collapse="") # flatten whatever structure it has
s <- gsub("[^a-zA-Z]", "", s)
if (noAmbig) {
ambCodes <- "([bjouxzBJOUXZ])" # parentheses capture the match
ambChar <- unlist(regmatches(s, regexec(ambCodes, s)))[1]
if (! is.na(ambChar)) {
stop(paste("Input contains ambiguous letter: \"", ambChar, "\"", sep=""))
}
}
if (uc) { s <- toupper(s)}
if (lc) { s <- tolower(s)}
return(s)
}
# ==== in2vec ==============================================
# Utility function to sanitize input and expand it into a
# vector of single characters. Arguments for in2seq are
# passed through via the three-dots parameter syntax.
in2vec <- function(s, ...) {
s <- in2seq(s, ...)
return(unlist(strsplit(s, "")))
}
# ==== addToDB =============================================
# Add a new protein entry to the database, with associated
# taxonomy entry
addToDB <- function(database,
name = "",
refseq_id = "",
uniprot_id = "",
taxonomy_id,
genome_xref = numeric(),
genome_from = numeric(),
genome_to = numeric(),
sequence = "",
species_name = "") {
if (missing(database)) {
stop("\"database\" argument is missing with no default.")
}
if (missing(taxonomy_id)) {
stop("taxonomy_id argument is missing with no default.")
}
if (! is.numeric(taxonomy_id)) {
stop(paste("taxonomy_id \"",
taxonomy_id,
"\" is not numeric. Please correct.", sep=""))
}
# check taxonomy_id
if (! any(database$taxonomy$id == taxonomy_id)) { # new taxonomy_id
if (missing(species_name)) {
stop(paste("taxonomy_id",
taxonomy_id,
"is not yet in database, but species_name",
"is missing with no default."))
}
else {
# add this species to the taxonomy table
database$taxonomy <- rbind(database$taxonomy,
data.frame(id = taxonomy_id,
species_name = species_name,
stringsAsFactors = FALSE))
}
}
# handle protein
# pid is 1 if the table is empty, max() + 1 otherwise.
if (is.null(nrow(database$protein))) { pid <- 1 }
else {pid <- max(database$protein$id) + 1}
database$protein <- rbind(database$protein,
data.frame(id = pid,
name = name,
refseq_id = refseq_id,
uniprot_id = uniprot_id,
taxonomy_id = taxonomy_id,
genome_xref = genome_xref,
genome_from = genome_from,
genome_to = genome_to,
sequence = in2seq(sequence),
stringsAsFactors = FALSE))
return(database)
}
# ==== setDB ===============================================
# Update database values
setDB <- function(database,
table,
id = NULL,
name = NULL,
refseq_id = NULL,
uniprot_id = NULL,
taxonomy_id = NULL,
genome_xref = NULL,
genome_from = NULL,
genome_to = NULL,
sequence = NULL,
species_name = NULL) {
if (missing(database) | missing(table)) {
stop("Database or table is missing with no default.")
}
if (table == "protein") {
if (is.null(id)) {
stop("Protein id is missing with no default.")
}
row <- which(database$protein$id == id)
if (! is.null(name)) { database$protein[row, "name"] <- as.character(name) }
if (! is.null(refseq_id)) { database$protein[row, "refseq_id"] <- as.character(refseq_id) }
if (! is.null(uniprot_id)) { database$protein[row, "uniprot_id"] <- as.character(uniprot_id) }
if (! is.null(taxonomy_id)) {
# must be numeric ...
if (! is.numeric(taxonomy_id)) {
stop(paste("taxonomy_id",
taxonomy_id,
"is not numeric. Please correct."))
}
# must exist in taxonomy table ...
if (! any(database$taxonomy$id == taxonomy_id)) { # new taxonomy_id
stop(paste("taxonomy_id",
taxonomy_id,
"not found in taxonomy table. Please update taxonomy table and try again."))
}
# all good, update it...
database$protein[row, "taxonomy_id"] <- taxonomy_id
}
if (! is.null(genome_xref)) { database$protein[row, "genome_xref"] <- genome_xref}
if (! is.null(genome_from)) { database$protein[row, "genome_from"] <- genome_from}
if (! is.null(genome_to)) { database$protein[row, "genome_to"] <- genome_to}
if (! is.null(sequence)) { database$protein[row, "sequence"] <- in2seq(sequence)}
}
else if (table == "taxonomy") {
if (missing(taxonomy_id)) {
stop("taxonomy_id is missing with no default.")
}
if (! any(database$taxonomy$id == taxonomy_id)) {
stop(paste(" Can't set values for this taxonomy_id.",
taxonomy_id,
"was not found in taxonomy table."))
}
row <- which(database$taxonomy$id == taxonomy_id)
if (species_name != "") { database$taxonomy[row, "species_name"] <- species_name }
}
else {
stop(paste("This function has no code to update table \"",
table,
"\". Please enter a valid table name."))
}
return(database)
}
# ==== getDBid =============================================
# Get a vector of IDs from a database table from all rows
# for which all of the requested attributes are true.
# Note: if no restrictions are entered, ALL ids are returned.
# We don't have code to select from genome coordinates, or
# query from sequence.
getDBid <- function(database,
table,
name = NULL,
refseq_id = NULL,
uniprot_id = NULL,
taxonomy_id = NULL,
species_name = NULL) {
if (missing(database) | missing(table)) {
stop("Database or table is missing with no default.")
}
if (table == "protein") {
sel <- rep(TRUE, nrow(database$protein)) # initialize
if (! is.null(name) ) { sel <- sel & database$protein[, "name"] == name }
if (! is.null(refseq_id) ) { sel <- sel & database$protein[, "refseq_id"] == refseq_id }
if (! is.null(uniprot_id) ) { sel <- sel & database$protein[, "uniprot_id"] == uniprot_id }
if (! is.null(taxonomy_id)) { sel <- sel & database$protein[, "taxonomy_id"] == taxonomy_id }
sel <- db$protein$id[sel] # get ids by selecting from vector
}
else if (table == "taxonomy") {
sel <- rep(TRUE, nrow(database$taxonomy)) # initialize
if (! is.null(taxonomy_id) ) { sel <- sel & database$taxonomy[, "id"] == taxonomy_id }
if (! is.null(species_name)) { sel <- sel & database$taxonomy[, "species_name"] == species_name }
sel <- db$taxonomy$id[sel] # get ids by selecting from vector
}
else {
stop(paste("This function has no code to select from table \"",
table,
"\". Please enter a valid table name."))
}
return(sel)
}
# ==== getSeq ==============================================
# Retrieve the sequences for given id matches from the
# protein table. Uppercase, to make Biostrings happy.
getSeq <- function(database, ...) {
if (missing(database)) {
stop("Database argument is missing with no default.")
}
ids <- getDBid(database, table= "protein", ...)
seq <- db$protein[ids, "sequence"]
return(toupper(seq))
}
# ==== MESSAGE ============================================
cat("db_utilities.R has been loaded. The following functions are now available:\n")
cat(" createDB()\n")
cat(" addToDB()\n")
cat(" setDB()\n")
cat(" getDBid()\n")
cat(" getSeq()\n")
cat(" in2seq()\n")
cat(" in2vec()\n")
cat(" \n")
# ==== TESTS =============================================
# TBD
# [END]
Save dbUtilities.R and source()
it to make the functions immediately available. They will also be available when you next start R.
source(paste(PROJECTDIR, "dbUtilities.R", sep=""))
We now have a first set of somewhat credible database functions. Let's create a database and add two proteins.
db <- createDB()
db <- addToDB(db,
name = "Mbp1",
refseq_id = "NP_010227",
uniprot_id = "P39678",
taxonomy_id = 4932,
genome_xref = "NC_001136.10",
genome_from = 352877,
genome_to = 355378,
sequence = "
1 msnqiysary sgvdvyefih stgsimkrkk ddwvnathil kaanfakakr trilekevlk
61 ethekvqggf gkyqgtwvpl niakqlaekf svydqlkplf dftqtdgsas pppapkhhha
121 skvdrkkair sastsaimet krnnkkaeen qfqsskilgn ptaaprkrgr pvgstrgsrr
181 klgvnlqrsq sdmgfprpai pnssisttql psirstmgpq sptlgileee rhdsrqqqpq
241 qnnsaqfkei dledglssdv epsqqlqqvf nqntgfvpqq qssliqtqqt esmatsvsss
301 pslptspgdf adsnpfeerf pgggtspiis miprypvtsr pqtsdindkv nkylsklvdy
361 fisnemksnk slpqvllhpp phsapyidap idpelhtafh wacsmgnlpi aealyeagts
421 irstnsqgqt plmrsslfhn sytrrtfpri fqllhetvfd idsqsqtvih hivkrksttp
481 savyyldvvl skikdfspqy rielllntqd kngdtalhia skngdvvffn tlvkmgaltt
541 isnkegltan eimnqqyeqm miqngtnqhv nssntdlnih vntnnietkn dvnsmvimsp
601 vspsdyityp sqiatnisrn ipnvvnsmkq masiyndlhe qhdneikslq ktlksisktk
661 iqvslktlev lkesskdeng eaqtnddfei lsrlqeqntk klrkrliryk rlikqkleyr
721 qtvllnklie detqattnnt vekdnntler lelaqeltml qlqrknklss lvkkfednak
781 ihkyrriire gtemnieevd ssldvilqtl iannnknkga eqiitisnan sha
",
species_name = "Saccharomyces cerevisiae")
db <- addToDB(db,
name = "Res2",
refseq_id = "NP_593032",
uniprot_id = "P41412",
taxonomy_id = 4896,
genome_xref = "NC_003424.3",
genome_from = 686543,
genome_to = 689179,
sequence = "
1 maprssavhv avysgvevye cfikgvsvmr rrrdswlnat qilkvadfdk pqrtrvlerq
61 vqigahekvq ggygkyqgtw vpfqrgvdla tkykvdgims pilsldideg kaiapkkkqt
121 kqkkpsvrgr rgrkpsslss stlhsvnekq pnssisptie ssmnkvnlpg aeeqvsatpl
181 paspnallsp ndntikpvee lgmleapldk yeeslldffl hpeegripsf lyspppdfqv
241 nsvidddght slhwacsmgh iemiklllra nadigvcnrl sqtplmrsvi ftnnydcqtf
301 gqvlellqst iyavdtngqs ifhhivqsts tpskvaaaky yldcilekli siqpfenvvr
361 lvnlqdsngd tslliaarng amdcvnslls ynanpsipnr qrrtaseyll eadkkphsll
421 qsnsnashsa fsfsgispai ispscsshaf vkaipsissk fsqlaeeyes qlrekeedli
481 ranrlkqdtl neisrtyqel tflqknnpty sqsmenlire aqetyqqlsk rlliwlearq
541 ifdlerslkp htslsisfps dflkkedgls lnndfkkpac nnvtnsdeye qlinkltslq
601 asrkkdtlyi rklyeelgid dtvnsyrrli amscginped lsleildave ealtrek
",
species_name = "Schizosaccharomyces pombe")
Now for YFO. Copy one of the samples above, edit it for the your Mbp1 homologue in YFO and add it to the database.
Then save the database, delete it and reload it:
save(db, file="proteinDB.RData") # write to file
rm(db) # remove
db # it's gone
load("proteinDB.RData") # read it back
db # verify
When that is done, we're ready to run some alignments.
Optimal Sequence Alignment at EMBOSS
Online programs for optimal sequence alignment are part of the EMBOSS tools. The programs take FASTA files or raw text files as input.
Local optimal sequence alignment using "water"
Task:
- Fetch the sequences for
MBP1_SACCE
andMBP1_YFORG
from your database. Something like:
getSeq(db, refseq_id = "NP_010227")
- Access the EMBOSS Explorer site (if you haven't done so yet, you might want to bookmark it.)
- Look for ALIGNMENT LOCAL, click on water, paste your sequences and run the program with default parameters.
- Study the results. You will probably find that the alignment extends over most of the protein, but does not include the termini.
- Considering the sequence identity cutoff we discussed in class (25% over the length of a domain), do you believe that the N-terminal domains (the APSES domains) are homologous?
- Change the Gap opening and Gap extension parameters to high values (e.g. 30 and 5). Then run the alignment again.
- Note what is different.
Global optimal sequence alignment using "needle"
Task:
- Look for ALIGNMENT GLOBAL, click on needle, paste the
MBP1_SACCE
andMBP1_YFORG
sequences again and run the program with default parameters. - Study the results. You will find that the alignment extends over the entire protein, likely with long indels at the termini.
The Mutation Data Matrix
The NCBI makes its alignment matrices available by ftp. They are located at ftp://ftp.ncbi.nih.gov/blast/matrices - for example here is a link to the BLOSUM62 matrix[2].
Scoring matrices are also available in the Bioconductor Biostrings package.
if (!require(Biostrings, quietly=TRUE)) {
source("https://bioconductor.org/biocLite.R")
biocLite("Biostrings")
library(Biostrings)
}
help(package = "Biostrings")
data(package = "Biostrings")
data(BLOSUM62)
BLOSUM62
A R N D C Q E G H I L K M F P S T W Y V B J Z X *
A 4 -1 -2 -2 0 -1 -1 0 -2 -1 -1 -1 -1 -2 -1 1 0 -3 -2 0 -2 -1 -1 -1 -4
R -1 5 0 -2 -3 1 0 -2 0 -3 -2 2 -1 -3 -2 -1 -1 -3 -2 -3 -1 -2 0 -1 -4
N -2 0 6 1 -3 0 0 0 1 -3 -3 0 -2 -3 -2 1 0 -4 -2 -3 4 -3 0 -1 -4
D -2 -2 1 6 -3 0 2 -1 -1 -3 -4 -1 -3 -3 -1 0 -1 -4 -3 -3 4 -3 1 -1 -4
C 0 -3 -3 -3 9 -3 -4 -3 -3 -1 -1 -3 -1 -2 -3 -1 -1 -2 -2 -1 -3 -1 -3 -1 -4
Q -1 1 0 0 -3 5 2 -2 0 -3 -2 1 0 -3 -1 0 -1 -2 -1 -2 0 -2 4 -1 -4
E -1 0 0 2 -4 2 5 -2 0 -3 -3 1 -2 -3 -1 0 -1 -3 -2 -2 1 -3 4 -1 -4
G 0 -2 0 -1 -3 -2 -2 6 -2 -4 -4 -2 -3 -3 -2 0 -2 -2 -3 -3 -1 -4 -2 -1 -4
H -2 0 1 -1 -3 0 0 -2 8 -3 -3 -1 -2 -1 -2 -1 -2 -2 2 -3 0 -3 0 -1 -4
I -1 -3 -3 -3 -1 -3 -3 -4 -3 4 2 -3 1 0 -3 -2 -1 -3 -1 3 -3 3 -3 -1 -4
L -1 -2 -3 -4 -1 -2 -3 -4 -3 2 4 -2 2 0 -3 -2 -1 -2 -1 1 -4 3 -3 -1 -4
K -1 2 0 -1 -3 1 1 -2 -1 -3 -2 5 -1 -3 -1 0 -1 -3 -2 -2 0 -3 1 -1 -4
M -1 -1 -2 -3 -1 0 -2 -3 -2 1 2 -1 5 0 -2 -1 -1 -1 -1 1 -3 2 -1 -1 -4
F -2 -3 -3 -3 -2 -3 -3 -3 -1 0 0 -3 0 6 -4 -2 -2 1 3 -1 -3 0 -3 -1 -4
P -1 -2 -2 -1 -3 -1 -1 -2 -2 -3 -3 -1 -2 -4 7 -1 -1 -4 -3 -2 -2 -3 -1 -1 -4
S 1 -1 1 0 -1 0 0 0 -1 -2 -2 0 -1 -2 -1 4 1 -3 -2 -2 0 -2 0 -1 -4
T 0 -1 0 -1 -1 -1 -1 -2 -2 -1 -1 -1 -1 -2 -1 1 5 -2 -2 0 -1 -1 -1 -1 -4
W -3 -3 -4 -4 -2 -2 -3 -2 -2 -3 -2 -3 -1 1 -4 -3 -2 11 2 -3 -4 -2 -2 -1 -4
Y -2 -2 -2 -3 -2 -1 -2 -3 2 -1 -1 -2 -1 3 -3 -2 -2 2 7 -1 -3 -1 -2 -1 -4
V 0 -3 -3 -3 -1 -2 -2 -3 -3 3 1 -2 1 -1 -2 -2 0 -3 -1 4 -3 2 -2 -1 -4
B -2 -1 4 4 -3 0 1 -1 0 -3 -4 0 -3 -3 -2 0 -1 -4 -3 -3 4 -3 0 -1 -4
J -1 -2 -3 -3 -1 -2 -3 -4 -3 3 3 -3 2 0 -3 -2 -1 -2 -1 2 -3 3 -3 -1 -4
Z -1 0 0 1 -3 4 4 -2 0 -3 -3 1 -1 -3 -1 0 -1 -2 -2 -2 0 -3 4 -1 -4
X -1 -1 -1 -1 -1 -1 -1 -1 -1 -1 -1 -1 -1 -1 -1 -1 -1 -1 -1 -1 -1 -1 -1 -1 -4
* -4 -4 -4 -4 -4 -4 -4 -4 -4 -4 -4 -4 -4 -4 -4 -4 -4 -4 -4 -4 -4 -4 -4 -4 1
BLOSUM62["H", "H"]
BLOSUM62["L", "L"]
BLOSUM62["S", "T"]
BLOSUM62["L", "D"]
Task:
- Study this and make sure you understand what this table is, how it can be used, and what a reasonable range of values for identities and pairscores for non-identical, similar and dissimilar residues is. Ask on the mailing list in case you have questions.
Alignment with Biostrings
Biostrings has extensive functions for sequence alignments. They are generally well written and tightly integrated with the rest of Bioconductor's functions. There are a few quirks however: for example alignments won't work with lower-case sequences. This is why our getSeq()
changes sequences to uppercase.
# sequence are stored in AAstring objects
?AAString
seq1 <- AAString(getSeq(db, refseq_id = "NP_010227"))
seq2 <- AAString(getSeq(db, refseq_id = "NP_593032")) # use MBP1_YFORG instead!
?pairwiseAlignment
# global alignment with end-gap penalties is default.
ali1 <- pairwiseAlignment(
seq1,
seq2,
substitutionMatrix = "BLOSUM62",
gapOpening = 10,
gapExtension = 0.5)
writePairwiseAlignments(ali1)
# local alignment
ali2 <- pairwiseAlignment(
seq1,
seq2,
type = "local",
substitutionMatrix = "BLOSUM62",
gapOpening = 50,
gapExtension = 10)
writePairwiseAlignments(ali2)
Task:
Have a look at the two alignments. Compare. The local alignment is weighted heavily to an indel-free alignment by setting very high gap penalties. Try changing them and see what happens.
BLAST
BLAST is by a margin the most important computational tool of molecular biology. It is so important, that we have already used BLAST in Assignment 2 even before properly introducing the algorithm and the principles, to find the most similar sequence to MBP1_SACCE
in YFO.
In this part of the assignment we will use BLAST to perform Reciprocal Best Matches.
One of the important questions of model-organism based inference is: which genes perform the same function in two different organisms. In the absence of other information, our best guess is that these are the two genes that are mutually most similar. The keyword here is mutually. If MBP1_SACCE
from S. cerevisiae is the best match to RES2_SCHPO
in S. pombe, the two proteins are only mutually most similar if RES2_SCHPO
is more similar to MBP1_SACCE
than to any other S. cerevisiae protein. We call this a Reciprocal Best Match, or "RBM"[3].
The argument is summarized in the figure on the right: genes that evolve under continuos selective pressure on their function have relatively lower mutation rates and are thus more similar to each other, than genes that undergo neo- or sub- functionalization after duplication.
Proteins are often composed of multiple domains that represent distinct roles in a gene's function. Under the assumptions above we could hypothesize:
- a gene in YFO that has the "same" function as the Mbp1 cell-cycle checkpoint switch in yeast should be an RBM to Mbp1;
- a gene that binds to the same DNA sites as Mbp1 should have a DNA-binding domain that is an RBM to the DNA binding domain of Mbp1.
Thus we'll compare RBMs in YFO for full-length Mbp1_SACCE
and its DNA-binding domain, and see if the results are the same.
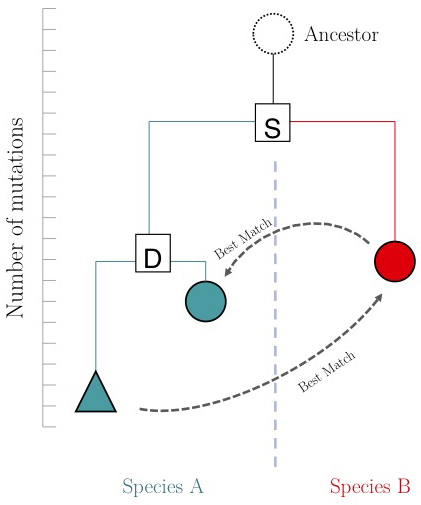
Full-length RBM
You have already performed the first half of the experiment: matching from S. cerevisiae to YFO. The backward match is simple.
Task:
- Access BLAST and follow the link to the protein blast program.
- Enter the refseq ID for
MBP1_YFORG
in the Query sequence field. - Select
refseq_protein
as the database to search in, and enterSaccharomyces cerevisiae (taxid:4932)
to restrict the organism for which hits are reported. - Run BLAST. Examine the results.
If your top-hit is NP_010227
, you have confirmed the RBM between Mbp1_SACCE
and Mbp1_YFORG
. If it is not, let me know. I expect this to be the same and would like to verify your results if it is not.
RBM for the DNA binding domain
The DNA-binding domain of Mbp1_SACCE
is called an APSES domain.
Defining the domain sequence
The APSES domain is a well-defined type of DNA-binding domain that is ubiquitous in fungi and unique in that kingdom. Structurally it is a member of the Winged Helix-Turn-Helix family. Recently it was found that it is homologous to the somewhat shorter, prokaryotic KilA-N domain; thus the APSES domain was retired from pFam and instances were merged into the KilA-N family. However InterPro has a KilA-N entry but still recognizes the APSES domain.
KilA-N domain boundaries in Mbp1 can be derived from the results of a CDD search with the ID 1BM8_A (the Mbp1 DNA binding domain crystal structure). The KilA-N superfamily domain alignment is returned.
- (pfam 04383): KilA-N domain; The amino-terminal module of the D6R/N1R proteins defines a novel, conserved DNA-binding domain (the KilA-N domain) that is found in a wide range of proteins of large bacterial and eukaryotic DNA viruses. The KilA-N domain family also includes the previously defined APSES domain. The KilA-N and APSES domains may also share a common fold with the nucleic acid-binding modules of the LAGLIDADG nucleases and the amino-terminal domains of the tRNA endonuclease.
10 20 30 40 50 60 70 80
....*....|....*....|....*....|....*....|....*....|....*....|....*....|....*....|
1BM8A 16 IHSTGSIMKRKKDDWVNATHILKAANFAKaKRTRILEKEVLKETHEKVQ---------------GGFGKYQGTWVPLNIA 80
Cdd:pfam04383 3 YNDFEIIIRRDKDGYINATKLCKAAGETK-RFRNWLRLESTKELIEELSeennvdkseiiigrkGKNGRLQGTYVHPDLA 81
90
....*....|....
1BM8A 81 KQLA----EKFSVY 90
Cdd:pfam04383 82 LAIAswisPEFALK 95
Note that CDD and SMART are not consistent in how they apply pFam 04383
to the Mbp1 sequence. See annotation below.
The CDD KilA-N domain definition begins at position 16 of the 1BM8 sequence. But virtually all fungal APSES domains have a longer, structurally defined, conserved N-terminus. Blindly applying the KilA-N domain definition to these proteins would lose important information. For most purposes we will prefer the sequence spanned by the 1BM8_A structure. The sequence is given below, the KilA-N domain is coloured dark green. By this definition the APSES domain is 99 amino acids long and comprises residues 4 to 102 of the NP_010227
sequence.
10 20 30 40 50 60 70 80
....*....|....*....|....*....|....*....|....*....|....*....|....*....|....*....|
1BM8A 1 QIYSARYSGVDVYEFIHSTGSIMKRKKDDWVNATHILKAANFAKAKRTRILEKEVLKETHEKVQGGFGKYQGTWVPLNIA 80
90
....*....|....*....
1BM8A 81 KQLAEKFSVYDQLKPLFDF 99
- Yeast APSES domain sequence in FASTA format
>APSES_MBP1 Residues 4-102 of S. cerevisiae Mbp1 QIYSARYSGVDVYEFIHSTGSIMKRKKDDWVNATHILKAANFAKAKRTRI LEKEVLKETHEKVQGGFGKYQGTWVPLNIAKQLAEKFSVYDQLKPLFDF
- Synopsis of ranges
Domain | Link | Length | Boundary | Range (Mbp1) | Range (1BM8) |
KilA-N: pfam04383 (CDD) | CDD alignment | 72 | STGSI ... KFSVY | 21 - 93 | 18 - 90 |
KilA-N: pfam04383 (SMART) | Smart main page | 79 | IHSTG ... YDQLK | 19 - 97 | 16 - 94 |
KilA-N: SM01252 (SMART) | Smart main page | 84 | TGSIM ... DFTQT | 22 - 105 | 19 - 99... |
APSES: Interpro IPR003163 | (Interpro) | 130 | QIYSA ... IRSAS | 3 - 133 | 1 - 99... |
APSES (1BM8) | – | 99 | QIYSA ... PLFDF | 4 - 102 | 1 - 99 |
Executing the forward search
Task:
- Access BLAST and follow the link to the protein blast program.
- Forward search:
- Enter only the APSES domain sequence for
MBP1_YFORG
in the Query sequence field (copied from above). - Select
refseq_protein
as the database to search in, and enter the correct taxonomy ID for YFO. - Run BLAST. Examine the results.
- If this is the same protein you have already seen, oK. If it's not add it to your protein database.
- Enter only the APSES domain sequence for
Alignment to define the sequence for the reverse search
Task:
- Define the YFO best-match APSES sequence by performing a global, optimal sequence alignment of the yeast domain with the full length protein sequence of your BLAST hit. Align these two sequences of very different length without end-gap penalties. Here is sample code that you can adapt.
# Align the yeast Mbp1 APSES domain with another protein sequence.
# Pattern:
apses <- AAString(in2seq("QIYSARYSGVDVYEFIHSTGSIMKRKKDDWVNATHILKAANFAKAKRTRI
LEKEVLKETHEKVQGGFGKYQGTWVPLNIAKQLAEKFSVYDQLKPLFDF"))
# Query:
# (Obviously, use the YFO best hit sequence instead of SCHPO...)
blastHit <- AAString(getSeq(db, refseq_id = "NP_593032"))
# This alignment uses the "overlap" type. "overlap" turns the
# end-gap penalties off and that is crucially important since
# the sequences have very different length.
aliApses <- pairwiseAlignment(
apses,
blastHit,
type = "overlap",
substitutionMatrix = "BLOSUM62",
gapOpening = 10,
gapExtension = 0.5)
# Inspect the result. The aligned sequences should be clearly
# homologous, and have (almost) no indels. The entire "pattern"
# sequence from QIYSAR ... to ... KPLFDF should be matched
# with the "query".
writePairwiseAlignments(aliApses)
# If this is correct, you can extract the matched sequence from
# the alignment object. The syntax is a bit different from what
# you have seen before: this is an "S4 object", not a list. No
# worries: as.character() returns a normal string.
as.character(aliApses@subject)
Executing the reverse search
Task:
- Copy the the APSES domain sequence for the YFO best-match and enter it into Query sequence field of the BLAST form.
- Select
refseq_protein
as the database to search in, and enterSaccharomyces cerevisiae (taxid:4932)
to restrict the organism for which hits are reported. - Run BLAST. Examine the results.
- Select
If your top-hit is again NP_010227
, you have confirmed the RBM between the APSES domain of Mbp1_SACCE
and Mbp1_YFORG
. If it is not, let me know. There may be some organisms for which the full-length and APSES RBMs are different and I would like to discuss these cases.
- TBC
Links and resources
Footnotes and references
- ↑ This is not strictly true in all cases: some algorithms measure similarity through an alignment-free approach, for example by comparing structural features, or domain annotations. However, these methods are mostly only important when sequences are so highly diverged that no meaningful alignment can be produced.
- ↑ That directory also contains sourcecode to generate the PAM matrices. This may be of interest if you ever want to produce scoring matrices from your own datasets.
- ↑ Note that RBMs are usually orthologues, but the definition of orthologue and RBM is not the same. Most importantly, many orthologues are not RBMs. We will explore this more when we discuss phylogenetic inference.
Ask, if things don't work for you!
- If anything about the assignment is not clear to you, please ask on the mailing list. You can be certain that others will have had similar problems. Success comes from joining the conversation.
- Do consider how to ask your questions so that a meaningful answer is possible:
- How to create a Minimal, Complete, and Verifiable example on stackoverflow and ...
- How to make a great R reproducible example are required reading.
< Assignment 3 | Assignment 5 > |